Data has become the lifeblood of modern healthcare, driving decisions from clinical treatments to operational planning. In 2025, the importance of data quality and data observability in healthcare cannot be overstated. For data professionals, decision-makers, and healthcare technology teams, ensuring accurate, complete, and reliable data is mission-critical. This blog will explore what data quality means in healthcare, why it matters for patient outcomes and efficiency, the consequences of poor data quality, and how next-generation solutions (like AI-driven data observability) are helping improve healthcare data. Real-world examples – from electronic health records (EHR) to insurance claims – will illustrate common challenges and the tangible impacts of data quality on patient care and decision-making.
What you will learn:
- What is Data Quality in Healthcare? – Key components of high-quality healthcare data and how it’s defined.
- Why Data Quality is Important in Healthcare – Benefits of quality data for patient safety, decision-making, operations, and compliance.
- Consequences of Poor Data Quality – Real-world scenarios where bad data causes inefficiencies, errors, or risks.
- How to Improve Healthcare Data Quality – Modern strategies and tools (including AI and data observability platforms) to maintain and enhance data quality.
- FAQ – Quick answers to common questions on data quality, causes of poor data, and improvement steps.
Let’s dive in and understand how better data quality is driving better healthcare.
What is Data Quality in Healthcare?
Data quality in healthcare refers to the accuracy, completeness, consistency, and timeliness of health-related data. This can include patient records, lab results, treatment plans, insurance claims, clinical trial data, and more. High-quality healthcare data means that information is correct, up-to-date, and formatted consistently, making it useful and trustworthy for decision-making.
Key dimensions of healthcare data quality:
- Accuracy: Are the data values correct? For example, a patient’s medication list should correctly reflect what they’re actually taking.
- Completeness: Is any critical data missing? A medical record should have all necessary fields (like allergies or past surgeries) filled in.
- Consistency: Do data values agree across sources? A patient’s name and ID should match across the EHR, pharmacy system, and lab reports.
- Timeliness: Is the data up-to-date and available when needed? Lab results or diagnostic images need to be recorded and accessible promptly for care decisions.
- Validity: Is the data in the correct format and range? For instance, blood pressure readings should be within realistic ranges and in the proper units.
In a healthcare context, data quality also means adhering to data governance standards and regulations. Standards like HL7 and FHIR ensure data is structured properly for exchange, and regulations like HIPAA mandate safeguarding patient information. Essentially, healthcare data quality is about having “fit-for-purpose” data – information that is correct and usable for clinical care, analytics, and compliance.
Why is Data Quality Important in Healthcare?
High-quality data is essential to effective care delivery, informed decision-making, and regulatory compliance. The importance of data quality in healthcare becomes especially clear when you consider how it influences everything from clinical decisions to executive strategies. Here’s how quality data impacts healthcare:
1. Better Clinical Decision-Making and Patient Outcomes (Patient Safety)
When clinicians have access to accurate and complete patient data, they can make better decisions that directly affect outcomes. Take something as simple as a missing allergy record in an EHR—this could lead to prescribing medication that triggers a severe reaction. Reliable data helps prevent such mistakes:
- Accurate Diagnoses: Quality data ensures symptoms, history, and test results are clearly recorded, enabling correct diagnoses.
- Effective Treatments: Providers rely on trustworthy data to avoid adverse drug interactions and unnecessary tests.
- Chronic Condition Management: Wearables and home-monitoring data must be reliable to support proper treatment adjustments.
A large hospital saw a 30% drop in medication errors after standardizing lab data in their EHR system. Accurate data is foundational to patient safety.
2. Enhanced Decision-Making for Healthcare Leaders
Executives and analysts need quality data to make sound decisions across the system. Without it, strategic plans and compliance efforts can go off course.
- Strategic Planning: Patient demographics and service use patterns help decide where to expand care or adjust staffing.
- Compliance and Policy: Reporting on infections, procedures, or quality metrics depends on clean, accurate data.
- Financial Accuracy: Errors in claims or billing data can cause delays, rejections, or revenue loss.
Poor-quality insurance claims data can cost millions. Clean data ensures reimbursements are timely and correct.
3. Operational Efficiency and Cost Control
Bad data slows operations, leads to waste, and increases workload:
- Avoiding Redundancy: Duplicate or inconsistent records can result in repeat tests, confusion, or missed follow-ups.
- Streamlining Systems: Standardized data enables smoother handoffs between departments and systems.
- Resource Use: Quality data improves scheduling, bed management, and equipment utilization.
One clinic found 15% of patient records were duplicates. Cleaning them up reduced unnecessary mailings and duplicate tests, improving care coordination and cutting costs.
4. Compliance and Reporting Accuracy
Regulatory requirements—from HIPAA to Medicare ratings—depend on high-quality data:
- Accurate Metrics: Hospitals must report quality indicators like readmission rates and infection stats with precision.
- Audit Readiness: Regulators expect consistency between reports and records.
- Trustworthy Scores: Programs like Medicare star ratings rely on clean data to assess provider performance.
In short, data quality underpins everything—from bedside care to executive decisions and compliance. In healthcare, better data isn’t just helpful—it’s critical.
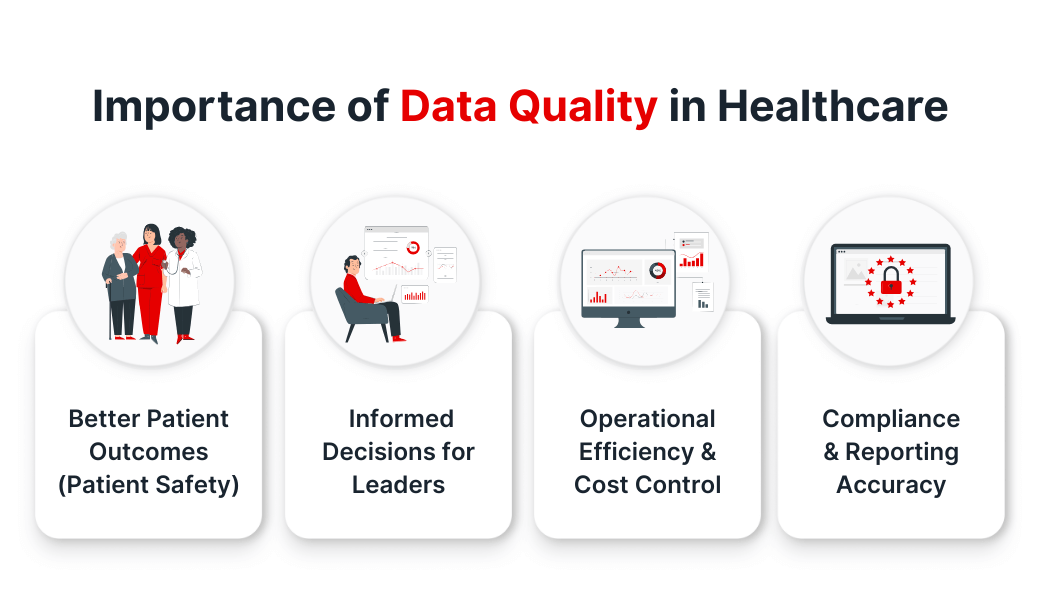
Consequences of Poor Data Quality in Healthcare
Understanding what goes wrong when data quality slips makes it clear just how important it is to get it right. Poor healthcare data quality compromises the accuracy of patient records, leading to adverse clinical outcomes and operational inefficiencies. In healthcare, bad data doesn’t just slow things down—it can put lives at risk.
Patient Safety Risks: If a patient’s allergy isn’t recorded or lab results are tied to the wrong record, the consequences can be dangerous. One small data error can lead to the wrong diagnosis, treatment delays, or worse.
Patient Frustration and Trust Issues: When patients are asked to repeat information or redo tests because of misplaced or inaccurate data, it’s not just inconvenient—it’s demoralizing. Repeated missteps erode trust and delay care.
Staff Burnout and System Distrust: Doctors and staff often spend hours fixing issues—resolving duplicates, chasing missing info, or working around unreliable systems. Over time, this wears them down and reduces trust in the tools meant to help them.
Operational Disruptions: A wrong insurance code can delay billing. A scheduling error might double-book an operating room or leave a team idle. These inefficiencies pile up fast.
Financial Fallout: From denied claims and compliance penalties to lawsuits and rework, the financial hit from poor data quality runs deep. Industry estimates point to billions lost each year.
Flawed Analytics, Misguided Decisions: AI and analytics are only as good as the data behind them. Incomplete or inaccurate data skews insights, misguides interventions, and impacts public health decisions at scale.
In short, poor data quality affects every layer of healthcare—from individual outcomes to system-wide efficiency.
How to Improve Data Quality in Healthcare (and the Role of Data Observability)
Improving data quality in healthcare isn’t a one-time initiative—it’s an ongoing effort that requires the right processes, tools, and mindset. Here are practical ways to elevate and maintain high data quality.
1. Establish Data Governance and Standards
Begin with a solid data governance framework. Define how data should be collected, entered, and shared. Standardize formats (e.g., MM/DD/YYYY for dates), abbreviations, and codes (like ICD-10). A governance committee can guide these efforts and ensure consistency across departments.
Tip: Train frontline staff—registration clerks, nurses, lab techs—on the importance of accurate data entry. Simple actions, like verifying two patient identifiers, can prevent duplicate records and reduce downstream errors.
2. Data Profiling and Auditing Regularly
Use data profiling to scan systems like EHRs or billing platforms for anomalies—null values, invalid codes, or duplicate records. Profiling can highlight issues like a sudden spike in missing lab results, pointing to technical or process gaps.
Supplement this with regular audits. Quarterly reviews of patient records, for example, can uncover incomplete or incorrect data in fields like allergies or medications, helping teams fix root causes.
3. Implement Automated Data Cleansing
Automated tools help correct large volumes of bad data efficiently. These tools can standardize terms (e.g., “Calif.” to “California”) and validate entries using reference data. In healthcare, this improves contact accuracy and ensures clean insurance or drug information.
Matching algorithms can spot likely duplicates—such as “Jane Doe” and “Janet Doe” at the same address—and merge records accordingly. Systems that validate against external sources (e.g., insurance databases or drug dictionaries) help reduce errors at the source.
4. Leverage Data Observability and Real-Time Monitoring
Data observability platforms continuously monitor data flows and alert teams when something breaks—like a drop in lab feed volume or a rise in default values (e.g., birthdates logged as 01/01/YYYY). These tools help catch issues early, before they impact care decisions.
They also ensure data consistency across systems. If an address is updated in one system but not another, observability tools can flag the mismatch, preserving integrity across the ecosystem.
Platforms like DQLabs.ai integrate with EHRs and other healthcare data sources to surface anomalies, predict future issues, and offer a live view of data quality across the organization.
5. Encourage Cross-System Integration and a Single Source of Truth
Fragmentation is a key cause of poor data quality. Integrate systems using standards like FHIR and maintain a Master Patient Index (MPI) to assign a unique ID to every patient. This reduces duplicates and keeps updates synchronized across systems like lab, pharmacy, imaging, and billing.
6. Data Quality Dashboards and KPIs
Track KPIs like completeness of contact fields, number of duplicate records, or time to resolve data issues. Dashboards help visualize department-level performance—for example, ER entries may lag behind radiology due to the fast-paced environment. Tools like DQLabs offer data trust scores and trend tracking to inform decisions.
7. Continuous Improvement and Culture
Encourage feedback from clinicians and frontline staff. Identify recurring issues—like inconsistent allergy documentation—and resolve the root cause. Celebrate improvements by linking them to better outcomes, such as improved model accuracy or fewer readmissions.
How DQLabs.ai Helps Improve Data Quality in Healthcare
As healthcare data complexity grows, specialized tools like DQLabs.ai provide an intelligent way to manage data quality at scale. While this blog isn’t a pitch, it’s worth noting how modern data quality platforms can streamline many steps above. DQLabs.ai, for example, offers capabilities well-suited for healthcare organizations aiming for higher data quality:
- AI-Powered Data Profiling: DQLabs continuously profiles healthcare datasets (EHR records, claims, research data, etc.) to assess quality. It uses AI to understand patterns and anomalies, automatically flagging issues like a sudden increase in missing values or a field that usually contains numeric lab results now having text errors. This smart profiling goes beyond rule-based checks, catching issues humans might overlook.
- Automated Data Cleansing & Enrichment: The platform can automatically correct common errors. For instance, if “New York” is misspelled in multiple ways across records, DQLabs can standardize them. It can also enrich data – for example, adding standardized medical codes or validating addresses – to improve completeness and consistency. These automated fixes save time for IT teams and ensure more uniform data across the board.
- Real-Time Data Monitoring (Data Observability): DQLabs provides a real-time observability layer, monitoring data pipelines. If a data feed from a medical device integration fails or starts delivering strange values (like a heart rate of 0 due to a device glitch), the system will trigger alerts. Real-time monitoring means issues are caught and resolved before they propagate and affect clinical decisions.
- Data Quality Dashboards and Scorecards: For decision-makers, DQLabs offers dashboards showing a “Data Quality Score” for different domains (patient data, financial data, etc.). A compliance officer could see at a glance if patient records meet quality thresholds, or a data manager could track improvement over time. It’s an easy way to communicate data health to stakeholders without diving into technical details.
- Integration & Collaboration: DQLabs integrates with popular healthcare data systems (cloud data warehouses, analytics platforms) and supports collaborative workflows. Data stewards and analysts can review and approve suggested fixes, add context to data issues, and jointly resolve quality problems. This collaboration ensures that data quality isn’t siloed – it becomes a shared responsibility with transparency.
By using a platform like DQLabs.ai, healthcare organizations can accelerate their journey toward high-quality data. It automates heavy lifting, provides intelligent insights, and lets teams focus on patient care and innovation rather than constantly firefighting data issues. In essence, DQLabs.ai acts as an “extra pair of eyes” on your data 24/7, which is especially valuable in the fast-paced, high-stakes world of healthcare.
Conclusion
Data quality in healthcare is not just a technical concern – it’s fundamentally tied to patient health, operational excellence, and strategic success. In 2025, as healthcare becomes even more data-driven with telemedicine, AI diagnostics, and personalized medicine, the integrity of data will directly impact quality of care and innovation.
Let’s recap the key takeaways:
- Patient Impact: High-quality data leads to safer, more effective patient care. Every data point – from a correct allergy entry to a complete surgical history – can influence clinical outcomes.
- Decision-Making: Healthcare leaders and AI models rely on data. Quality data empowers confident, accurate decisions whether in a board meeting or by an algorithm in the ER. Poor data misguides those decisions with potentially harmful consequences.
- Efficiency & Cost: Clean, consistent data streamlines operations, reduces redundant work, and cuts costs by avoiding mistakes and rework. In a climate of rising healthcare costs, data quality improvements translate to saved time and money.
- Compliance & Trust: Regulators and patients alike expect data to be right. Trust in a healthcare system hinges on its information being accurate and private. Data quality underpins compliance efforts and maintains the institution’s reputation.
- Continuous Effort: Maintaining data quality is an ongoing journey, blending people, process, and technology. Through good governance, regular audits, training, and modern tools like AI-driven data observability, healthcare organizations can achieve a state of continuous data excellence.
Ultimately, focusing on data quality is focusing on healthcare quality. By treating data as a critical asset – as important as the doctors, devices, and drugs in delivering care – we ensure that healthcare organizations are data-driven for the better, not data-burdened by errors.
Investing in data quality pays dividends in better patient outcomes, streamlined operations, and breakthrough insights that shape the future of health. In a world where data is the new heartbeat of healthcare, keeping that heartbeat strong and healthy is everyone’s responsibility.
FAQs on Healthcare Data Quality
Q1. How can healthcare organizations improve data quality?
Healthcare organizations can improve data quality by implementing strong data governance, using standards for data entry (like standardized coding for diagnoses), and training staff on accurate data capture. Regular data audits and data profiling help identify errors or gaps. Investing in automated data cleansing tools can fix common issues (e.g., remove duplicates, correct formatting). Many organizations are also adopting data observability platforms (often AI-driven, such as DQLabs.ai) to continuously monitor data flows and catch anomalies in real time. The key is to make data quality an ongoing priority: measure it, assign responsibility (data stewards or a data governance team), and use technology to maintain it proactively.
Q2. What are the main causes of poor data quality in healthcare systems?
Several common issues contribute to poor healthcare data quality:
- Human Error: In busy settings like ERs, mistakes during data entry—typos, wrong dropdowns, or misunderstood forms—are frequent and compound over time.
- Disconnected Systems: Lab, pharmacy, billing, and clinical tools often don’t talk to each other. Without integration, updates get missed or data becomes inconsistent.
- No Common Standards: Without standardized formats, the same condition might appear as “Hypertension,” “HBP,” or just a code—confusing systems and teams.
- Outdated IT Systems: Older platforms may not enforce rules, allowing blanks, invalid entries, or inconsistent formats into the record.
- Mergers & Migrations: Combining systems during hospital mergers or EHR upgrades can introduce duplicates, misaligned fields, or lost data.
- Limited Training: When staff aren’t trained in data quality or user interfaces are unclear, workarounds emerge—like dumping details into free-text fields.
Q3. Why is data quality truly important in healthcare (in one summary)?
Because lives depend on it. Accurate healthcare data helps providers treat patients safely, make informed decisions, and avoid costly or harmful errors. It supports better care, smoother operations, and compliance with regulations. For example, if an allergy or medication is missing from a patient’s record, it could result in a dangerous interaction. Good data also powers reliable analytics, billing, and public health reporting. Poor data, on the other hand, creates risks—from misdiagnoses to waste and mistrust. Reliable data is the backbone of effective, safe, and scalable healthcare.
Q4. How does data observability differ from traditional data quality checks?
Traditional checks are often periodic and rule-based—like scripts that flag missing fields. Data observability is real-time, continuous, and system-wide:
- It monitors live data flows for issues like missing files or schema changes.
- It detects anomalies using metrics and patterns—even without predefined rules.
- AI/ML helps spot issues faster, reducing manual rule writing.
- It offers lineage to trace problems back to their source.
Think of it as a real-time guardrail for your data, complementing static checks with dynamic insights.
Q5. Can you give examples of data quality issues in electronic health records (EHRs)?
Absolutely. EHRs are rich in information—but prone to quality issues that affect care and reporting:
- Duplicate Records: A patient may have multiple records due to name variations—like “John Smith” and “Jon Smith”—leading to split histories for meds or allergies.
- Missing or Wrong Info: Key fields like allergies or current meds may be blank or outdated, especially after system migrations.
- Free-Text Inconsistencies: Typos or abbreviations (e.g., “insuline” or “Htn”) in notes can confuse both clinicians and analytics tools.
- Coding Mistakes: Small code errors can be serious—“D64.9” (anemia) vs. “E64.9” (nutrition issue) changes the diagnosis completely.
- Outdated Sections: One part of a record may show an active prescription, while another lists it as discontinued—often due to missed updates during transitions of care.
- Formatting Mismatches: Units like mg/dL vs. mmol/L in lab data can cause dangerous misreads if not standardized across systems.
These issues show why continuous, system-wide data quality efforts are essential—not just for care, but also for research, billing, and policy.