How to Navigate Alert Fatigue with DQLabs
How to Navigate Alert Fatigue with DQLabs https://www.dqlabs.ai/wp-content/uploads/2024/07/thumbnail-6-1024x575.webp 1024 575 DQLabs DQLabs https://www.dqlabs.ai/wp-content/uploads/2024/07/thumbnail-6-1024x575.webpAlert fatigue refers to a situation in which a high volume of alerts and notifications overwhelm people to a state where they tend to ignore the alerts. This is obviously not a good scenario! The whole idea of having alerts and notifications mechanism is to detect and resolve outlier issues. Alert fatigue is increasingly becoming a common phenomenon in the data quality monitoring space. In this article, we’ll delve into the risks, impacts, and mechanisms to deal with it.
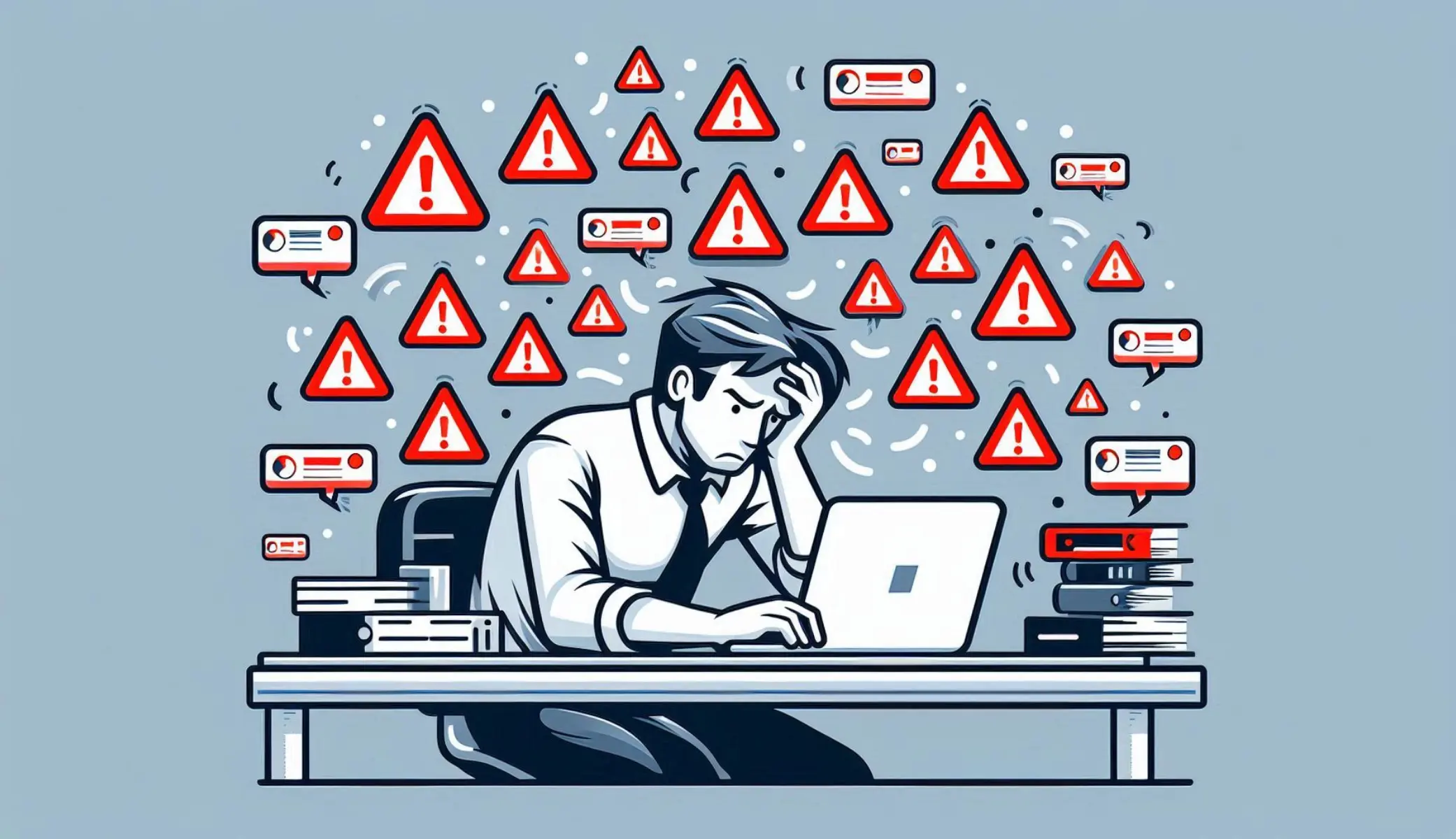
Reasons for alert fatigue
Before we think about how to deal with alert fatigue, we need to understand what are different reasons that cause alert fatigue:
- Volume of alerts: If your data quality and monitoring tools provided alerts for everything, it would become impossible for you to address every alert. The sheer volume of alerts is one of the most common reasons why data teams become desensitized to alerts. It goes without saying that if any major data quality issues go unnoticed it can cause some serious problems.
- Lack of incident resolution: Alerts without a proper incident resolution process increase the time taken to address the data quality issues. If every member of your team is notified of every alert, the team may start overlooking issues, assuming that the responsibility for their resolution belongs to another individual. Data teams may also find themselves resolving each issue on a case-to-case basis. Without a proper incident resolution process, data teams would have to understand each data quality error in detail. The lack of a process certainly affects issue ownership, timely resolution and of course, data teams’ productivity.
- No alert prioritization mechanisms: One of the major reasons for alert fatigue is the absence of prioritization mechanisms based on alert severity. If everything is urgent and important then nothing is urgent or important! If your data user is notified 30-40 times a day, with multiple alerts, ranging from a minor alert (“Backup to cloud complete”) to a high-priority one (“Your sales data pipeline has paused data ingestion”), what are the chances that he would identify the most important one among the 40 he has received all day?
Impact of alert fatigue
- Lack of responsiveness: When data teams find themselves drowning in a sea of alerts, it’s natural for them to start ignoring alerts completely. The risks of this can be multifold – data users’ response time to critical data alerts increases issue resolution time and restricts the data consumption by downstream users, lowering their productivity. This increases what we know as “data downtime“, which has risks of its own. What’s worse is if the alerts go noticed and do not get addressed at all, the consequences of “bad data” can be far more intense & even more difficult to resolve.
- Decreased efficacy: Alert fatigue creates a lot of issues in downstream data consumption. Users may be waiting around for issues to get resolved, or sometimes may not know that certain issues have gone unnoticed and may find out much later – at the visualization stage of data consumption.
- Lost productivity: Many errors go unnoticed due to the ignorance driven by alert fatigue. This leads to a reactive approach to dealing with alerts which makes the data quality issues resolution process more time-consuming. One data issue might have led to a few others and now data teams need to start resolving more data quality issues. This forces data teams to spend their valuable time fixing the data quality issue, when teams could have spent it on much more productive tasks.
- Loss of trust: Ever-increasing alerts, and the fatigue caused by them, negatively impact the data quality issues that lead to higher data downtime. This affects the timely consumption of accurate and reliable data by downstream data and business users. Eventually, data and business users lose trust in their data system which affects data-driven decision-making.
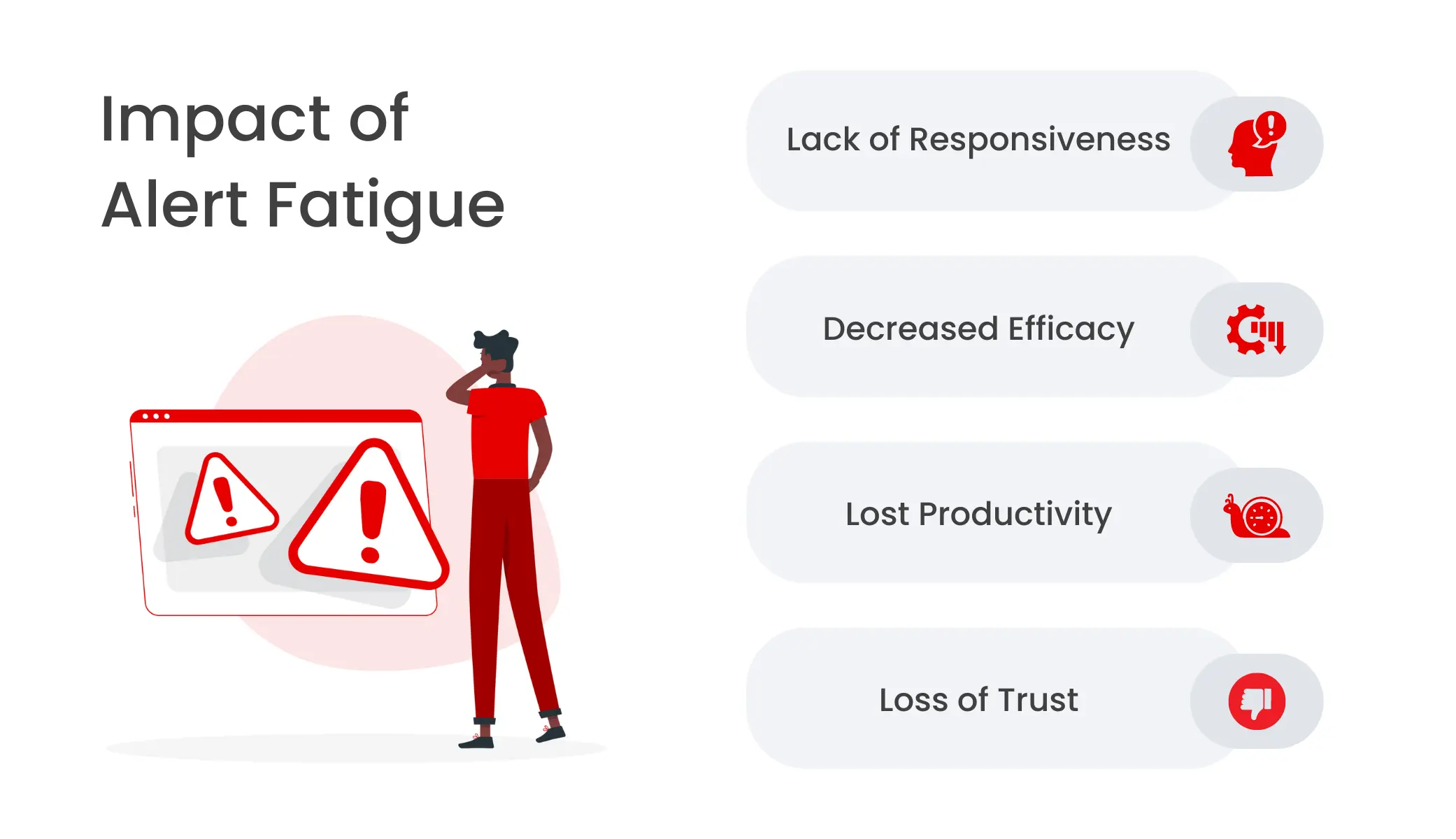
How to manage alert fatigue
Organizations need to follow certain practices to effectively manage alerts and reduce alert fatigue to address data quality issues proactively.
Alert categorization: Alerts are of no importance if you don’t know the severity of them. Organizations need data quality tools that categorize alerts based on their severity to empower data users to effectively manage and address alerts and address data quality issues.
Threshold setting: Data teams need data quality tools that can provide functionality to select the threshold for their data quality issues. By customizing alerts based on the threshold users can decrease the alert generation and efficiently address the alerts.
AI and ML-driven alerts: Data quality tools that generate AI and ML-driven data quality alerts streamline the process of anomaly detection. Leveraging AI and ML, these tools automatically detect any deviation of data from the baseline scenario and based on the amount of deviation provide severity of alerts.
Evaluation window configuration: The Evaluation window refers to the period (number of days, weeks, etc) in which your data quality tools monitor your data patterns. For example, if you know that your data changes significantly every Thursday (compared to the previous day) it leads to a lot of alert generation every Thursday. In this case, you need to change your evaluation window from a daily frequency to a weekly frequency which will reduce your alert fatigue significantly.
Reduce alert fatigue with DQLabs
DQLabs, the Modern Data Quality Platform, empowers users with AI/ML-driven anomaly detection and alert prioritization to reduce organizational alert fatigue. DQLabs’ anomaly detection mechanism detects an expected value range of data flow based on historical trends and calculates the deviation from the benchmark. If the actual value is different from the expected value range the platform provides a deviation and if the deviation is not in the expected value range it will trigger the alert. The unique value proposition here is that based on the deviation percentage, DQLabs will automatically set the priority of the alert (High, medium, or low), this will help users to only focus on the priority alerts and start addressing the alerts accordingly.
Benefits enabled by DQLabs’ anomaly detection
Alerts prioritization
With the AI & ML enabled anomaly detection process, DQLabs automates the process of data quality issues tracking. Based on the deviation from the standard data, DQLabs set the priority of the alerts (high, medium, and low).
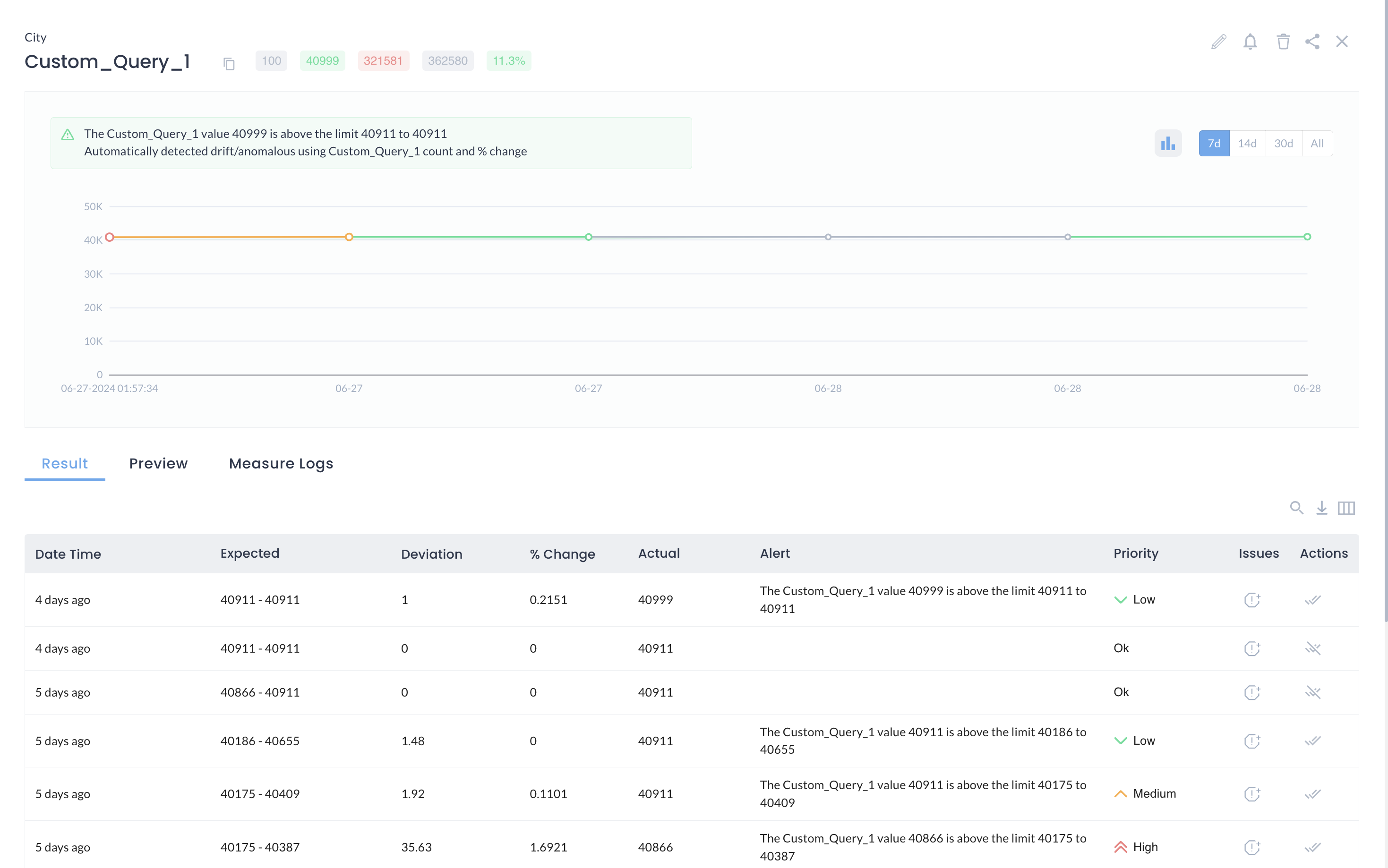
Alerts customization
DQLabs’ anomaly detection capability is not limited to the AI & ML-based default configuration. DQLabs empowers users to select the manual threshold as per their specific dataset requirements. For example, if the row count deviation is 20% but users are okay with up to 35% deviation. Users can manually set the deviation as 35%. Now users won’t get unnecessary alerts which will dramatically reduce their alert fatigue.
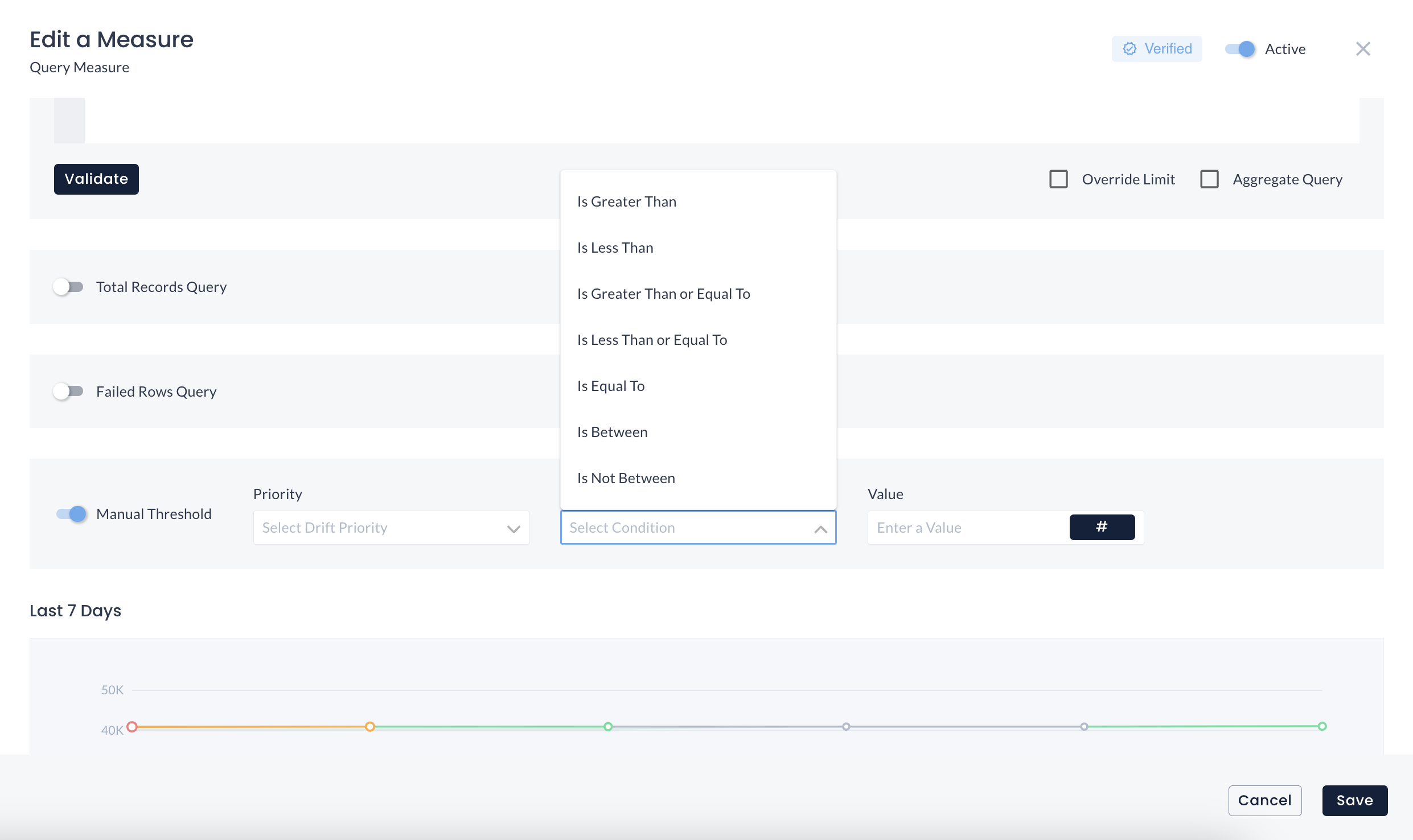
Issue resolution process
After the alerts are generated, users can check the severity of alerts in the DQLabs platform. Based on that, users can assign these alerts to relevant personas. DQLabs provides a mechanism to alert users in their most preferred issue management tools and communication channels like Jira, Slack, and BigPanda.
Evaluation window configuration
DQLabs empowers users with the ability to configure the minimum number of runs to measure the forecasting of their data patterns. For example, users can choose the minimum run as 5 (if their data changes periodically every 5 days), due to this they won’t get any unnecessary alerts if there’s a significant deviation from the last day. This capability reduces unnecessary alerts significantly.
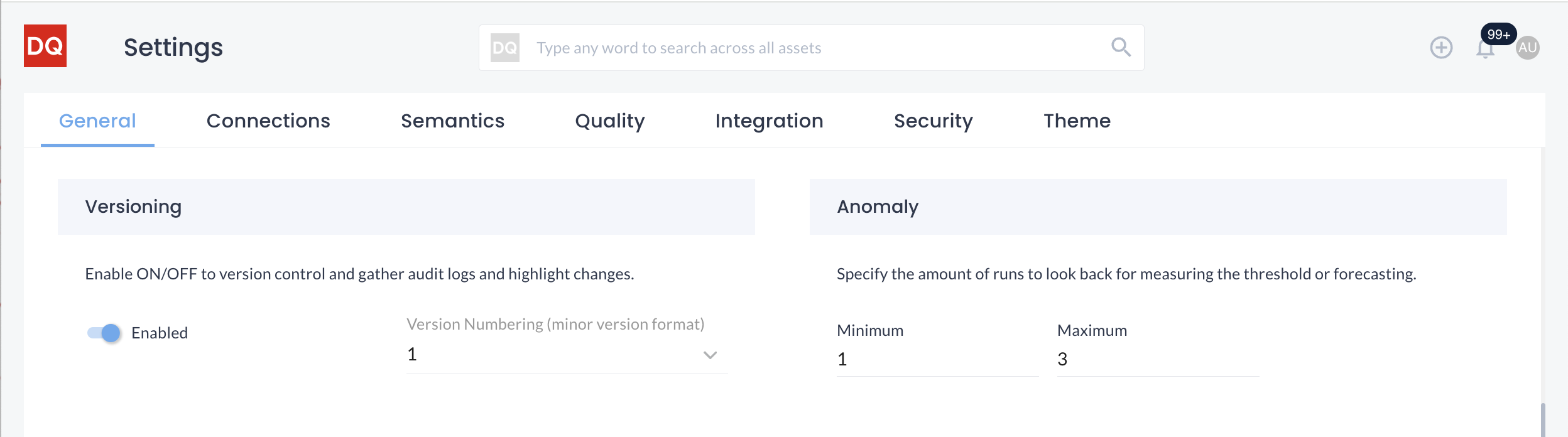
Conclusion
Managing alert fatigue is crucial for maintaining effective data quality monitoring and ensuring that critical issues are promptly addressed without overwhelming data teams. By implementing robust practices such as alert categorization based on severity, setting appropriate thresholds, and leveraging AI and ML-driven anomaly detection, organizations can significantly reduce the volume of alerts and prioritize those that require immediate attention. DQLabs offers advanced capabilities in automating alert prioritization and customization, allowing teams to focus only on high-priority alerts and optimize their incident response processes.
Furthermore, configuring evaluation windows and integrating with issue management platforms like Jira, Slack, and BigPanda enhances collaboration and efficiency in resolving data quality issues. These practices not only mitigate alert fatigue but also enhance productivity by minimizing the time spent on unnecessary alerts and fostering trust in data quality monitoring systems. Ultimately, by adopting these strategies, organizations can ensure their data remains reliable, actionable, and supports informed decision-making across all levels of the business.