What is Data Downtime and How to Navigate it with Data Observability
What is Data Downtime and How to Navigate it with Data Observability https://www.dqlabs.ai/wp-content/uploads/2024/06/thumbnail-data-pipeline-blog-1-1024x575.webp 1024 575 DQLabs DQLabs https://www.dqlabs.ai/wp-content/uploads/2024/06/thumbnail-data-pipeline-blog-1-1024x575.webpEver feel like your data initiatives are stuck in neutral? Are your reports missing crucial details or arriving too late to be useful? Do you feel your team doesn’t trust the accuracy of your organization’s data? You’re not alone. Many companies invest heavily in data-driven strategies but struggle to get reliable results. Why? The culprit might be data downtime. Haven’t heard of it? Surely, you must have experienced it. Let’s take a look at these examples:
- You head a team of data engineers, building a platform for an FMCG company to track and analyze the data for each of its businesses. In a senior-level meeting, when the marketing team presented revenue numbers to the CEO, to their embarrassment, it was pointed out by a senior member of the sales team that the data they were presenting was outdated – it was from last month.
- You are a data scientist at an e-commerce company, trying to predict demand for your bestseller, the men’s cotton shirt. While running your usual ML models on the given data, it threw up an error. On closer inspection, you realize that there was a mistake in the data format – new data on customer comments was recently added to the system to improve your ML model accuracy, which was ingested incorrectly. However, this wasn’t flagged at the source system, and now you have to painstakingly correct the data input manually.
If you have ever faced any of the above scenarios, you have been a victim of data downtime! But what exactly is data downtime, and how does it happen?
Data downtime refers to the period in which your data is incomplete, inaccurate, and inconsistent, or in layman’s terms, stale and unusable data, that affects the timely consumption of it by various downstream users, anyone from your team’s data scientist, all the way up to your leadership, or at the very worst case, your customer!
Reasons for increased data downtime
- Increased data adoption: Organizations around the world are empowering their teams with data. Everyone, right from the intern to the CEO, has access to various levels of data, and each stakeholder is creating, updating & consuming this data at an unprecedented pace. This empowerment of users is of course necessary for organizations to promote a data-driven culture, but the flip side is that this is creating & complicating the issue of data downtime. Data that is touched by more users has a higher probability of being inconsistent (the sales team updated the user data on their end, but it wasn’t updated in the master database, so the marketing team is still working with an older version) or even incorrect (typos during data entry)!
- Complexity of Modern data stack: The modern data stack has evolved so much in the past few years. A good one usually consists of many technical elements with the objective of achieving all aspects of curating & processing raw data for consumption by downstream analytics users. This includes many different elements including various data sources, data integration, data storage, data orchestration, and BI and Data analytics. This increases the complexity of data management, which inevitably has led to an increased risk of data downtime.
- Federated data ownership and data products: Organizations are also adopting federated data management to promote data democratization and self-service data management. This means that domain teams are now taking ownership of their data assets and treating their data as a product. For example, the Sales team creates & updates all the data around sales calls & latest estimates of sales volumes for the month. This data may be made available to users downstream in marketing, to check, say, conversion rates for an advertisement or a promotion. This brings various business teams closer and promotes a data-driven culture in organizations, but at the same time, it also creates data downtime issues. If the sales team’s data is not frequently or correctly integrated with the marketing team’s or there is a lack of interoperability of data assets, this would certainly lead to more data downtime issues.
- Lack of automation: To handle data quality issues some organizations are still relying on traditional tools that lack automation and require human intervention to tackle data quality and observability issues. This could very frequently cause human error in data and increased data downtime, especially if inexperienced users may be updating or consuming the data. Traditional data quality and observability tools also lack the capabilities of AI & ML driven data anomaly detection. This could actually let some severe data issues go undetected, and when teams eventually do end up detecting them it’s often too late.
Cost Implications of Data Downtime
Data downtime is proportional to data travel time
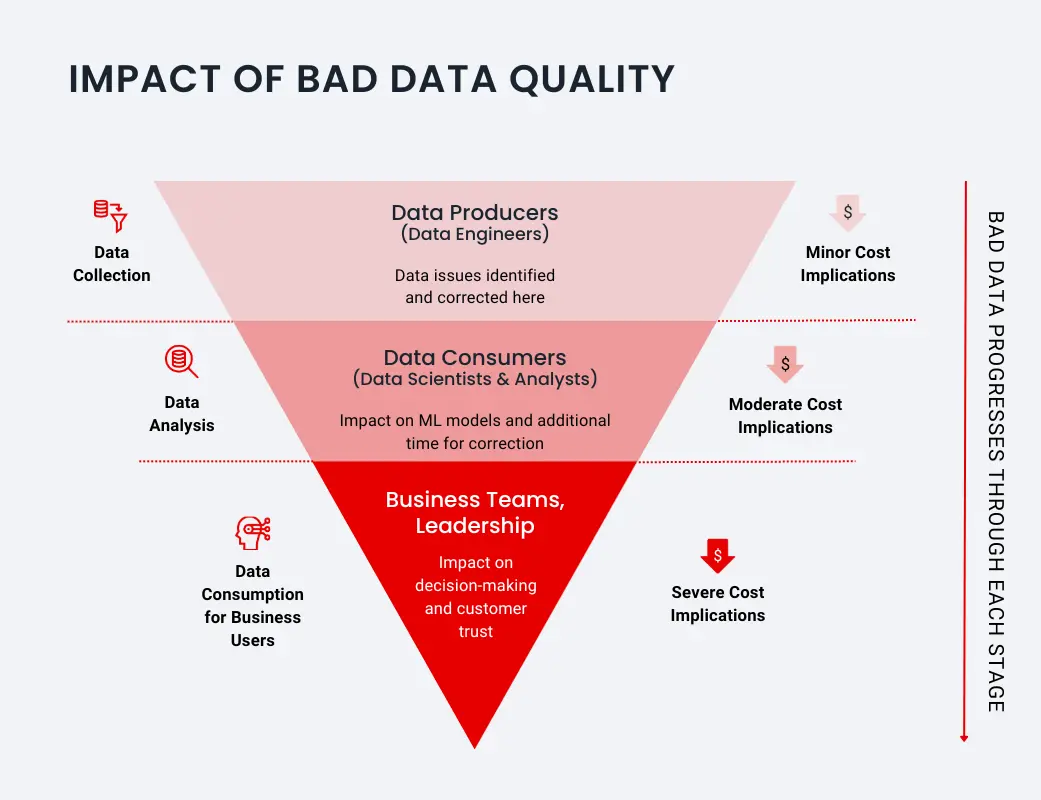
For simplicity, we can assume three sections of data stakeholders: data producers (data engineers), data consumers (data scientists, data analysts), business and leadership (CDOs, business teams, and most importantly, the customer!)
The cost of downtime is strongly associated with who identifies it! If the issues are found at the early stage (by engineers), the problem is easily addressable, but any later than that, and the cost implications can be severe.
For data consumers, the impact may be a loss of productivity. Data teams spend their valuable time in addressing and resolving data quality issues – time they could have spent on product innovation and more revenue-generating activities, like actually building a functioning ML model.
For a rough estimate of this, organizations can calculate productivity loss cost with the following formula:
Productivity loss = (10-30%)*Average salary of data scientist /data engineer* Total number of Data engineers and Scientists
The assumption here is that a typical data engineer loses 10-30% of his/her productivity to deal with data downtime issues.
For business teams & leadership, data downtime can have some dire consequences, especially if insights are drawn or decisions are made based on incorrect data. If an organization continues to face regular downtime issues, the employees will eventually start to lose trust in their data landscape and cause a huge dent in the data-driven culture of the organization.
Of course, if the consumer identifies data downtime issues, this could lead to anything from a minor inconvenience, all the way to loss of a brand’s trust & sometimes even regulatory challenges for incorrect information.
To summarize, the later you find the data issues the more is the cost implication of your data downtime incident.
Data elements that cause data downtime
We have seen organizational-level elements that play a role in increasing data downtime. But what about the data itself? There are certain data quality dimensions that are prime factors of data downtime, not restricted to:
- Missing values: Data that may be missing due to human errors or pipeline breakdown.
- Schema: When the structure of your data changes, like adding new fields or modifying existing ones.
- Freshness: How up-to-date is your data?
- Duplicates: Is the data unique and free from any duplicates?
- Completeness: Does the dataset contain all values from all relevant fields?
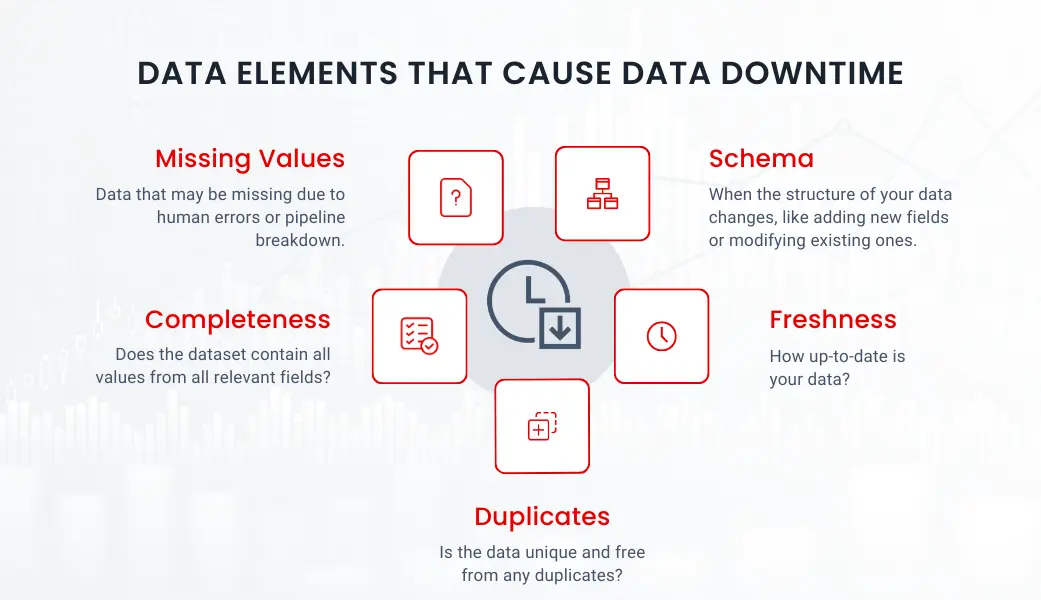
All these issues increase the data downtime.
Data observability as a solution for Data Downtime
We have discussed that the cost of data downtime can be very fatal for organizations but how can they resolve this issue? It is established that to truly decrease the cost implications of data downtime, organizations have to identify and resolve issues at the earliest. We saw how it was easier for a data engineer to troubleshoot data pipelines than for data scientists to change and work around their models with faulty data.
Data Observability is the perfect solution for this. It enables organizations to detect data issues at the earliest and to stop bad data from traveling throughout their organizational value chain.
Data observability refers to the ability to observe, monitor, and understand the behavior and performance of data systems in real-time. It encompasses the visibility into data pipelines, processes, and infrastructure, allowing organizations to ensure data reliability, quality, and availability.
Data observability empowers data teams to track and monitor data issues and provides a mechanism to identify and resolve issues before it’s too late. What’s even more interesting is that modern data observability tools, driven by AI and ML, provide automated alerts for every possible data anomaly. A data engineer no longer has to anticipate all the potential data-related challenges, and can automatically keep track of the ongoing ones. Modern Data Observability tools provide automatic alerts for any data anomalies that may arise, and prioritize those alerts based on their deviation from standard data flows, categorizing the severity of these alerts accordingly. This helps data teams to prioritize data issues and create remediation processes.
Why DQlabs
DQLabs offers a full stack Data Observability Solution as part of its Modern Data Quality Platform, tailored to meet the evolving needs of today’s enterprises. With DQLabs, organizations can proactively monitor and analyze their data pipelines, model performance, and potential biases in real-time and reduce the cost implications of data downtime. By leveraging automation, contextual insights, and advanced analytics, DQLabs empowers businesses to unlock the full potential of their data, driving informed decision-making and fostering AI-driven innovation at scale. Embrace the power of data observability with DQLabs and embark on a journey towards eliminating data downtime and promoting a data-driven culture.