Flawed data leads to inaccurate analysis and missed opportunities. As organizations handle more data than ever, data teams often struggle with ensuring data quality and integrity across all their systems. Without a structured approach, inconsistencies and errors can undermine business decisions and erode trust in data.
Achieving high data quality requires a clear strategy and consistent best practices. Without them, businesses risk making decisions based on incomplete or unreliable information. Understanding the key challenges and common pitfalls is the first step toward building a stronger data foundation.
In this blog, we outline a complete plan for data teams to enhance data quality—covering its importance, common challenges, and a step-by-step approach to improvement. This strategy emphasizes automation and data observability to ensure data remains accurate, consistent, and reliable. By following this plan, your team can implement a robust program that improves data quality and maintains data trust over the long term.
Why Is Data Quality Important?
As organizations scale and manage increasing volumes of data, ensuring data quality is more critical than ever. Poor data quality can lead to unreliable analytics, flawed AI models, and ineffective business decisions. Inconsistent, incomplete, or outdated data creates inefficiencies, reduces operational effectiveness, and erodes trust in data-driven insights.
By prioritizing data quality, businesses can improve efficiency, enhance customer experiences, and make more informed decisions. Despite its importance, many organizations continue to struggle with poor data quality due to factors like siloed systems, manual processes, and a lack of visibility into data issues. Understanding these challenges is the first step toward improving data quality and ensuring data-driven success.
Challenges With Data Quality
Regulatory Compliance
Organizations must navigate strict regulations like GDPR, CCPA, and HIPAA, requiring accurate, privacy-compliant data. Without strong data quality controls, businesses risk compliance failures and penalties. Ensuring data traceability and accuracy is critical for audits and regulatory reporting.
Limited Resources
Maintaining high data quality requires investment in tools, skilled personnel, and time. However, data teams often have limited budgets, making it difficult to allocate resources for continuous data cleansing and monitoring. As a result, issues like duplicates, missing values, and outdated records persist.
Inconsistent Data Across Systems
Data from multiple sources is often recorded in different formats or standards, leading to discrepancies. For instance, customer names, addresses, or product codes may not match across databases, causing errors in reporting and decision-making. Addressing inconsistencies requires standardizing data formats and integrating siloed systems.
Lack of Ownership
Without clear accountability, data issues remain unresolved. Many organizations lack designated owners responsible for data accuracy, leading to fragmented efforts and poor governance. Defining roles like data stewards ensures that someone is accountable for maintaining data integrity.
Resistance to Change
Introducing new data governance policies, automation tools, or quality controls often faces internal resistance. Employees may be reluctant to adopt new processes, preferring familiar workflows even if they result in poor data quality. Driving cultural change requires leadership support, training, and demonstrating quick wins.
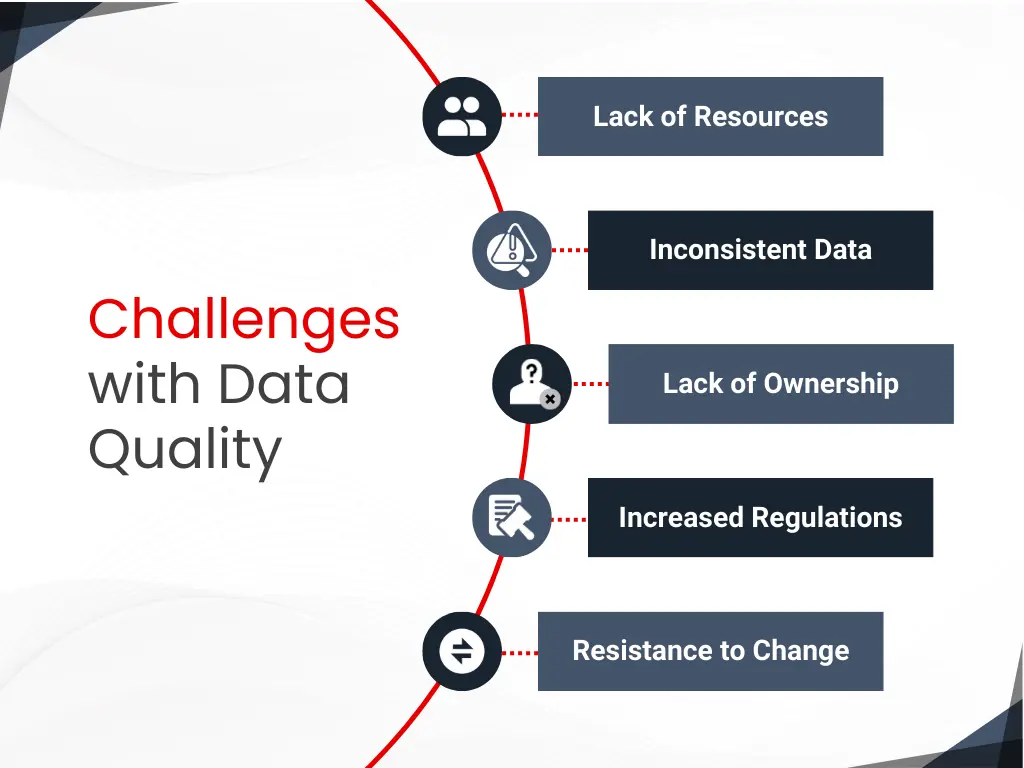
How to Improve Data Quality: A Step-by-Step Approach
If you’re wondering how to improve data quality across your organization, following a structured and repeatable approach is the key to long-term success.
Below is a step-by-step framework that can help you build and sustain high data quality across teams, systems, and processes.
Step 1: Define Goals and Objectives
Start by identifying key data quality issues affecting your organization, such as duplicate records, missing values, or inconsistent formatting. Set measurable goals like “Reduce duplicate records by 90% in six months” or “Ensure 98% completeness in customer data.” Clear objectives help align data quality initiatives with business priorities and provide benchmarks for measuring success.
Step 2: Get Stakeholder Support
Improving data quality is not just a technical effort—it requires buy-in across the organization. Secure support from executives, department heads, and key data users by demonstrating how high-quality data drives better decision-making, reduces inefficiencies, and ensures regulatory compliance.
Showcase the impact of poor data quality through real-world examples—such as revenue loss due to incorrect customer data or compliance risks from missing records. Highlighting these costs can help justify investment in data quality initiatives. When leadership prioritizes data quality, teams are more likely to follow best practices and adopt necessary tools.
Step 3: Assign Roles and Responsibilities
Data quality should be a shared responsibility, with clear ownership to ensure accountability. Establish a data governance structure that assigns specific roles for managing data quality. Designate data owners for key domains like Finance, Customer, and Product.
These individuals, typically from business units, understand the context of the data and are accountable for its accuracy and usage. They approve data definitions, resolve discrepancies, and ensure adherence to standards.
Supporting them, data stewards or data quality analysts handle daily data monitoring, cleansing, and validation tasks. Define who is responsible for reviewing reports, approving changes, and coordinating cleanup efforts. A structured governance model clarifies accountability and ensures continuous oversight.
Additionally, consider forming a data governance committee that meets regularly to discuss policies, emerging issues, and necessary improvements. By assigning well-defined roles, organizations create a culture where maintaining high data quality is a collective responsibility rather than an afterthought.
Step 4: Assess Current State and Set Target State
Before improving data quality, you need a clear understanding of your current state. Data profiling is the first step in the data quality management process, providing visibility into the structure, content, and quality of data. Conduct a data quality assessment using data profiling tools to detect errors, missing values, duplicates, and inconsistencies.
For example, if 20% of customer records lack an email address or dates appear in multiple formats, these need immediate attention.
In addition to technical profiling, gather insights from data users—business analysts, data engineers, and customer-facing teams—to understand real-world pain points. Their input helps identify usability and accuracy issues beyond what profiling tools detect.
Once the current state is mapped, define a target state with measurable KPIs. For instance, if 20% of records are incomplete, a realistic goal may be reducing that to below 5%. If inconsistencies exist across systems, standardizing formats should be a priority. This gap analysis (current vs. target) allows teams to prioritize improvements based on business impact, ensuring efforts are both strategic and measurable.
Step 5: Implement Your Data Quality Framework and Processes
With clear goals set, the next step is execution. Implement a data quality framework with a combination of remediation efforts and ongoing controls:
- Data Cleansing and Standardization: Correct inaccurate records, fill missing values, and eliminate duplicates. Standardize key fields like dates, addresses, and IDs to ensure uniformity across systems.
- Data Quality Rules and Validation: Embed preventive measures by setting validation rules. For instance, ensure email fields contain “@” or restrict date formats to a standard structure. Automated validation at data entry prevents errors from propagating.
- Master Data Management (MDM) and Integration: Create a single source of truth by reconciling duplicate or inconsistent records across systems. Proper integration ensures that updates in one system propagate accurately to others.
- Automation and Monitoring: Utilize data quality tools to automate profiling, cleansing, and validation tasks. Implement data observability to monitor pipelines in real time, detecting anomalies, delays, or broken feeds before they impact operations.
By embedding these processes, organizations ensure sustainable, high-quality data that fuels better decision-making.
Step 6: Evaluate and Improve Your Approach
Data quality is not a one-time project—it requires continuous evaluation. Track key data quality metrics through reports or dashboards. Are errors decreasing? Are business users experiencing fewer data-related issues? Regular monitoring ensures progress.
Solicit user feedback to uncover new challenges or improvements. Often, as major issues get resolved, smaller but critical ones emerge. Adjust your strategy by refining validation rules, adding automation, or providing additional training.
To sustain momentum, establish a quarterly review cycle where teams assess new risks and optimize existing processes. This iterative approach ensures that data quality continuously improves, even as business needs evolve.
Step 7: Create Data Advocacy Groups
Changing company-wide attitudes toward data quality requires cultural transformation. Form Data Quality Advocacy Groups—cross-functional teams of data champions who promote best practices within their departments.
Identify key individuals (e.g., Sales Ops, Finance, Marketing analysts) who regularly work with data and empower them to advocate for quality standards. These groups serve as liaisons between central data teams and business units, facilitating issue resolution at the source.
When employees see their peers prioritizing data quality, adoption rates improve organically. Over time, this peer-driven accountability fosters a data-driven culture where quality becomes second nature.
Step 8: Provide Data Quality Training Programs
Even with data quality automation and strict validation in place, human factors remain a major determinant of data quality. Conduct targeted training for different roles:
- Data Entry Teams: Teach proper data entry standards and emphasize the consequences of errors (e.g., how missing values affect reports and compliance).
- Data Stewards & Analysts: Train them on profiling techniques, data cleaning strategies, and quality monitoring tools.
- All Employees: Raise awareness of data quality’s business impact and encourage proactive issue reporting.
Training should be continuous—integrate it into onboarding, conduct regular refresher sessions, and provide bite-sized educational content through internal portals. A well-trained workforce is the key to sustaining long-term data quality improvements.
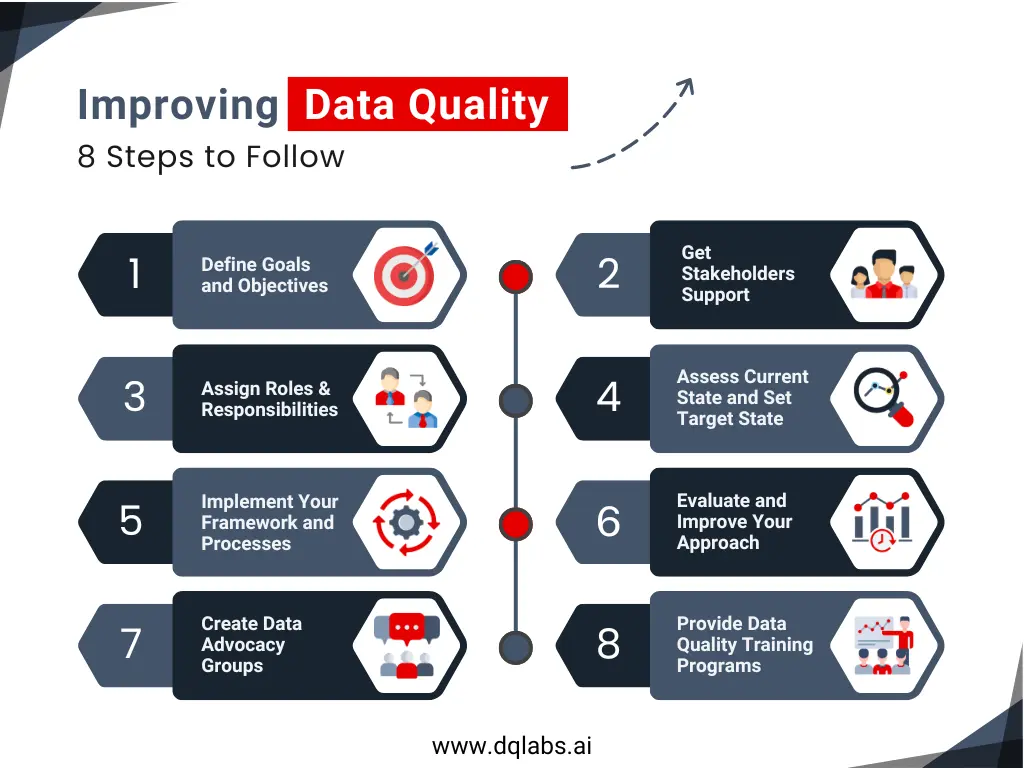
Conclusion
Improving data quality is an ongoing process, not a one-time fix. By setting clear objectives, securing stakeholder buy-in, and leveraging the right tools, organizations can build a solid foundation for reliable data. High-quality data strengthens analytics, reporting, and decision-making, driving better business outcomes.
Regularly revisiting data quality metrics and practices ensures adaptation to evolving challenges. While internal efforts help, modern data quality platforms like DQLabs accelerate success. As an AI-powered solution, DQLabs automates data profiling, anomaly detection, and issue resolution.
DQLabs also provides end-to-end data observability, alerting teams to issues before they escalate. With DQLabs, organizations enforce standards and improve data quality with minimal manual effort.
To achieve sustained data quality, define clear objectives, put the right processes in place, and empower teams with tools like DQLabs. The result? Data you can trust—fueling smarter decisions and business growth.
FAQs
Why is data quality important for businesses?
Data quality is crucial because businesses rely on accurate, complete, and reliable data for decision-making, customer interactions, and compliance. Poor data quality leads to incorrect insights, operational inefficiencies, regulatory risks, and lost revenue.
Consider the impact of inaccurate customer data: a simple mistake in a customer’s contact details can lead to failed communications, missed sales opportunities, or even compliance violations in regulated industries. In contrast, high-quality data ensures that decisions are based on facts rather than assumptions, improving efficiency and competitiveness.
To avoid these risks, organizations must take proactive steps to improve data quality through better processes, tools, and governance.
Beyond decision-making, data quality also affects automation, analytics, and AI models. Clean data fuels AI-driven insights, while poor data leads to biased models, incorrect predictions, and flawed automation. In financial services, for example, data errors can result in compliance breaches or inaccurate risk assessments. In healthcare, bad data can cause incorrect patient treatments or misdiagnoses.
Investing in data quality isn’t just about preventing errors—it’s about enabling business growth, innovation, and customer satisfaction.
What are the key dimensions of data quality?
Data quality is often evaluated across several key dimensions, including:
- Accuracy: The data correctly represents reality or the intended object. (For example, a customer’s recorded phone number is their actual current phone number.)
- Completeness: All required data is present. Nothing important is missing. (Every record has all the fields filled in where expected, such as every invoice having a date, customer name, and amount.)
- Consistency: Data is the same across applications and datasets. There are no conflicts or variations of the same data in different places. (If a product price is $100 in one system, it should also be $100 in another system, not $100 in one and $95 in another.)
- Timeliness (Freshness): Data is up-to-date and available when needed. (The information reflects the latest updates – e.g., inventory data shows the current stock levels, not last month’s.)
- Validity: Data is formatted and used in accordance with the rules and expectations. (Dates are in a valid date format, emails have a proper structure, numeric fields are within expected ranges, etc.)
- Uniqueness: Each real-world entity is recorded once in the dataset, avoiding duplicates. (A customer isn’t mistakenly entered into the database twice under slightly different names, which would create duplicate records.)
Focusing on these dimensions helps organizations pinpoint where data might be failing. For instance, data could be accurate but not timely, or complete but not consistent across systems. A robust data quality program aims to optimize all of these dimensions for its critical data assets.
How can automation improve data quality management?
Automation can significantly enhance data quality management by making it more efficient and consistent. Instead of relying solely on humans to find and correct data issues (which can be slow and error-prone), automated processes and tools take on much of the heavy lifting:
- Continuous Monitoring: Automated data quality tools can continuously scan datasets and data pipelines to detect anomalies or errors in real time. For example, an automated system can flag if today’s sales data load is 20% lower than usual (potentially indicating missing records) or if an unexpected null value appears in a field that should always have data. This immediate detection is something humans might not catch until much later. With built-in data pipeline observability, these tools also provide end-to-end visibility into data flow, helping teams quickly pinpoint where and why issues occur within complex data ecosystems.
- Validation and Enforcement: Automation can enforce data quality rules automatically. When new data is entered or integrated, automated validation scripts can check that it meets the required format and business rules, rejecting or quarantining bad data before it enters the main database. This prevents a lot of garbage data from ever accumulating.
- Data Cleansing and Deduplication: Automated processes can regularly clean the data – for instance, removing known invalid entries, standardizing formats (like converting all states to their abbreviations), and merging duplicate records. Using machine learning, some advanced tools can even predict and correct certain errors (such as fixing obvious typos or standardizing nicknames to legal names).
- Scalability: As data volume grows, manual data quality checking doesn’t scale well. Automation shines in this scenario; it can handle checking millions of records across dozens of quality rules without fatigue. This ensures data quality improvement efforts cover all your data, not just samples.
- Reduction of Human Error: By automating repetitive data handling tasks, you remove the risk of human mistakes in those tasks. People can then focus on higher-level analysis and management rather than tediously scrubbing data. When used strategically, automation becomes a powerful way to improve data quality at scale while reducing operational burden.
What challenges do data teams face in improving data quality?
Data teams often encounter several challenges when working to improve data quality:
- Securing Executive Buy-In: Convincing leadership to prioritize and invest in data quality can be difficult. If executives don’t see the immediate ROI, data quality projects may lack funding or urgency, leaving teams without the support needed to drive change.
- Resource Constraints: Many data teams are small or juggling multiple responsibilities. They may lack enough people or budget for dedicated data quality initiatives and tools. With limited resources, it’s challenging to tackle large-scale data cleanup or to continuously monitor data health.
- Data Silos and Inconsistencies: In many organizations, data is spread across different systems and departments that don’t communicate well. Each may have its own standards, causing inconsistencies (for example, one department might use full country names while another uses country codes). These silos make it tough to get a unified, accurate view of data and require significant effort in integration and standardization.
- Lack of Clear Ownership: If it’s not clearly defined who “owns” the quality of each dataset, then everyone assumes someone else will fix problems. This lack of accountability means issues linger. Data teams struggle when business users aren’t engaged in owning their data’s quality or when there’s no data governance structure assigning responsibility.
- Cultural Resistance: Introducing new data quality processes or asking people to change how they input/manage data can meet resistance. Some team members might not follow the new rules or might see data quality tasks as low priority. Overcoming the “we’ve always done it this way” mindset is a real challenge; it takes education and sometimes enforcement to change habits and get broad adoption of data quality practices.
- Measuring Impact: It can be hard to measure and demonstrate the improvement in data quality in terms that matter to the business. Data teams might implement fixes, but translating those into business KPIs (like revenue gains or cost savings) is not straightforward. Without clear metrics showing success, keeping momentum and justification for ongoing data quality work can be difficult.
How does data observability contribute to better data quality?
Data observability provides deep visibility into data health, allowing teams to proactively detect and resolve issues. It ensures:
- Early Detection of Anomalies – Identifies pipeline failures, missing records, or unusual trends.
- End-to-End Data Monitoring – Tracks data flow from source to destination, pinpointing problem areas.
- Automated Quality Checks – Ensures data meets accuracy, completeness, and consistency standards.
- Faster Incident Resolution – Integrates with alerting systems to notify teams of data issues before they impact business decisions.
- Continuous Improvement – Uses historical trends to strengthen data pipelines over time.
For example, a retail company using data observability can detect when sales data from one store isn’t syncing correctly with the central database. Instead of discovering the issue during financial reporting, the system alerts teams immediately, preventing revenue miscalculations.
By implementing data observability, organizations can move from reactive fixes to proactive data quality management.