How Data Quality Powers Effective Data Products
How Data Quality Powers Effective Data Products https://www.dqlabs.ai/wp-content/uploads/2024/06/thumbnail-data-pipeline-blog-5-1024x575.webp 1024 575 DQLabs DQLabs https://www.dqlabs.ai/wp-content/uploads/2024/06/thumbnail-data-pipeline-blog-5-1024x575.webpA few years ago, data used to be the hidden gem of the engineer’s toolbox. Neither is it the best-kept secret by the company’s founder. No longer is it confined to the auditor, the accountant or the lawyer’s computer. Today, leveraging data is part of almost every employee’s job description. Each employee consumes one or multiple data products at some point in their career, if not on a daily basis. But does that mean that she knows how to build, use or strategically leverage these data products?
Before we dive into their powerful abilities, let’s take a look at what data products really are.
Data products are consumption-ready datasets which are accurate, reliable, and trustworthy and which empower organizations to answer business-critical questions in a data-driven manner.
It doesn’t take our experts to tell you that data products are business-critical, but pay attention to the word “consumption ready”. Data, as we know, has little value in its raw form, however, once it is parsed through a series of data preparation steps (including ingestion, storage, integration, and curation), its power can be manifold.
While this technical definition of a data product may make it sound very niche, there is still a broader acceptance of what can be considered a data product. Going by the definition itself, any BI tool, enterprise data platform, enterprise LLM, or even an app that leverages data to answer specific business questions can be called a data product. But here’s the catch – in order to become a strategic asset, a true data product is one which is discoverable (easy for users to access), trustworthy (high data quality), secure and interoperable.
How to Deliver Successful Data Products
In order to successfully deliver data products an organization needs two key weapons in its arsenal. One is, an enterprise data management platform and the second, is domain-driven data ownership.
Enterprise Data Platform
As we have discussed earlier, in order to make your raw data ready for enterprise consumption, it needs to go through a series of data transformation steps. Starting from data ingestion and data storage, all the way to data curation and data orchestration, data needs to be “prepared” such that it can be leveraged by downstream users for their business and analytics use cases. Any good enterprise data platform has a collection of tools and key technologies that address these needs. This includes components of ETL pipelines, cloud data warehouses, data integration, data quality, data observability and governance capabilities, among others, to deliver timely and accurate data to various domains within an organization.
Say, for instance, you have a dashboard to visualize the important KPIs of various business units for your CEO. You should build an EDP that has the following capabilities –
Data Ingestion: The business team responsible for building the dashboard would provide a list of different data sources to the data team. Data engineers will build data pipelines to eventually create a centralized repository of all relevant data assets.
Data quality: A good EDP provides a mechanism for efficient data quality management with monitoring and alert mechanisms. This process ensures that the data goes through certain quality checks to ensure the data is accurate, fresh, and consistent.
Data transformation: Based on the specific requirements of the business team, data engineers transform data into specific data formats, ready to be consumed by business users.
Data access and governance: An EDP provides easy access to data scientists, data analysts, and business users in a self-serving manner to promote data democratization across the organization. EDP also enables the processes to implement data governance practices as per the organization’s policies.
Flexibility and scalability: A good EDP should be able to meet the organization’s growing data demands and, hence, it should come with in-built flexibility. This includes the ability to deal with different data sources, as well as different data types (structured, semi-structured, and unstructured) to enable a variety of business use cases.
With these capabilities, an EDP transforms raw data into consumption-ready datasets. However, building an enterprise data platform itself is not enough to successfully deliver data products, because every problem isn’t a data or a technology problem. Sometimes organizations must think about changing their perspective on how they treat their data…
Decentralized Domain-Driven Ownership
The answer lies in domain-driven data ownership. As per traditional data mesh principles, in a decentralized ownership model, domain teams such as marketing, sales, and HR, take complete ownership of their data, treating data with product management principles.
This includes taking care of accessibility, manageability and interoperability while managing the complete lifecycle of data products, just like in traditional product management. This decentralized approach empowers business teams to have complete autonomy and ownership over their data assets and enables them to align their data assets with their business objectives.
What does this really mean? Well, in order to successfully deliver data products that can drive domain-driven business decisions, what organizations need is simply a cross-functional team that comprises both data and business personas. Business personas would be responsible for defining the outcome and purpose of the data products. The data team, comprising data engineers, data scientists, and data stewards, would set up data infrastructure and enable data governance initiatives. The data product owner, the most important persona, would be responsible for the complete ownership of the data product and would find herself involved in the complete lifecycle of the product, right from inception/ideation to the retirement/discarding stage.
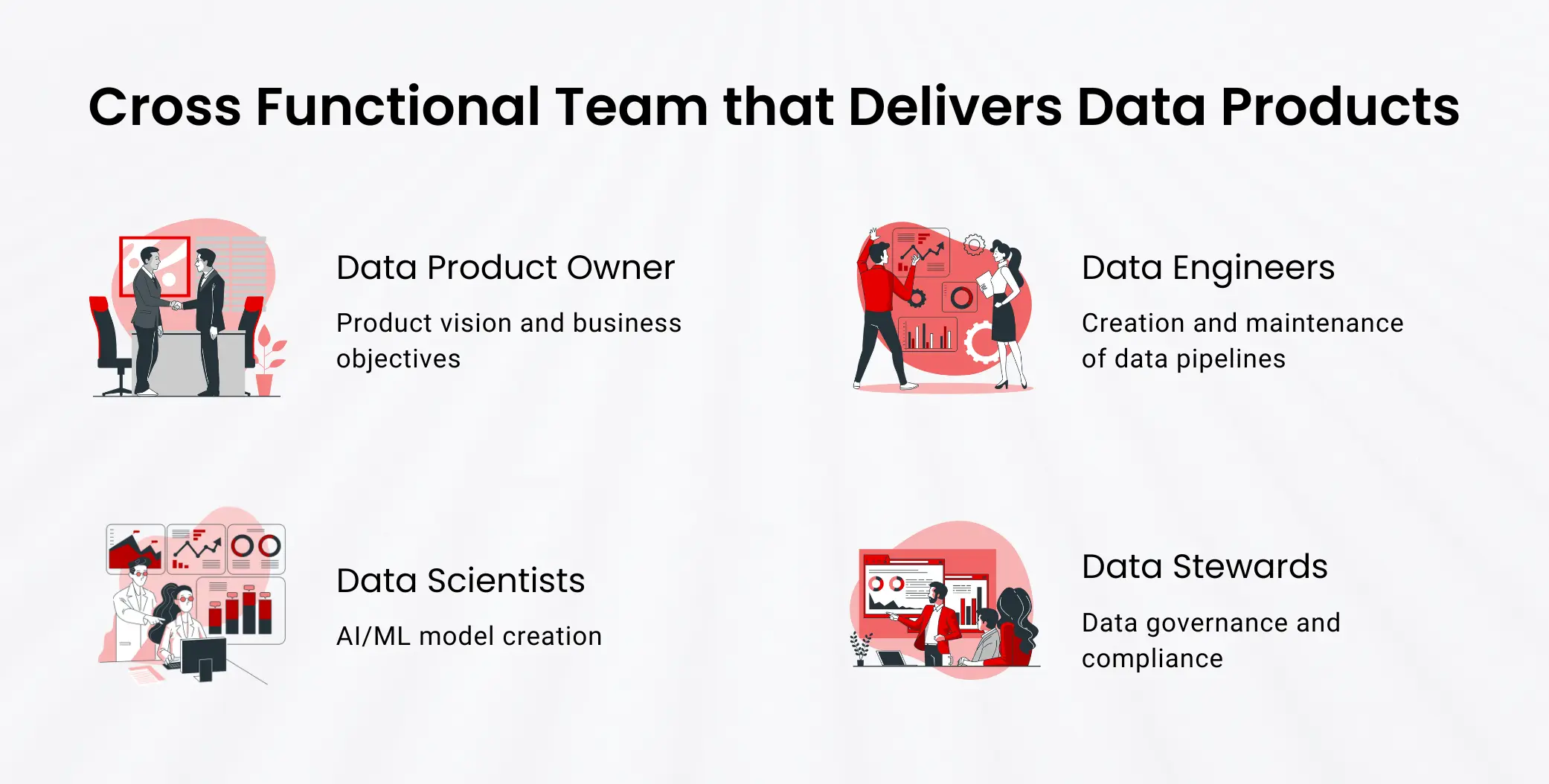
Characteristics of Good Data Products
Just like any traditional product, a data product has its own unique set of characteristics, and ensuring its availability is the sole responsibility of its data product owner!
Trusted Data: Your data products are as good as the data you feed into them. As they popularly say in the tech world, “Garbage in Garbage out!” Poor data quality can completely derail your data product initiatives and prevent you from being a data-driven organization. It is important to ensure that an organization’s data products are doing well on key data quality dimensions such as accuracy, completeness, consistency, timeliness, uniqueness, and validity.
Accessibility: Data products should be accessible to relevant user personas whenever they need them for consumption. It’s a data product owner’s responsibility to ensure that data products are accessible to relevant users and teams across the organization.
Secure and compliant: Data product owners must ensure that their data products meet all the security, governance, and compliance requirements set at the organization level.
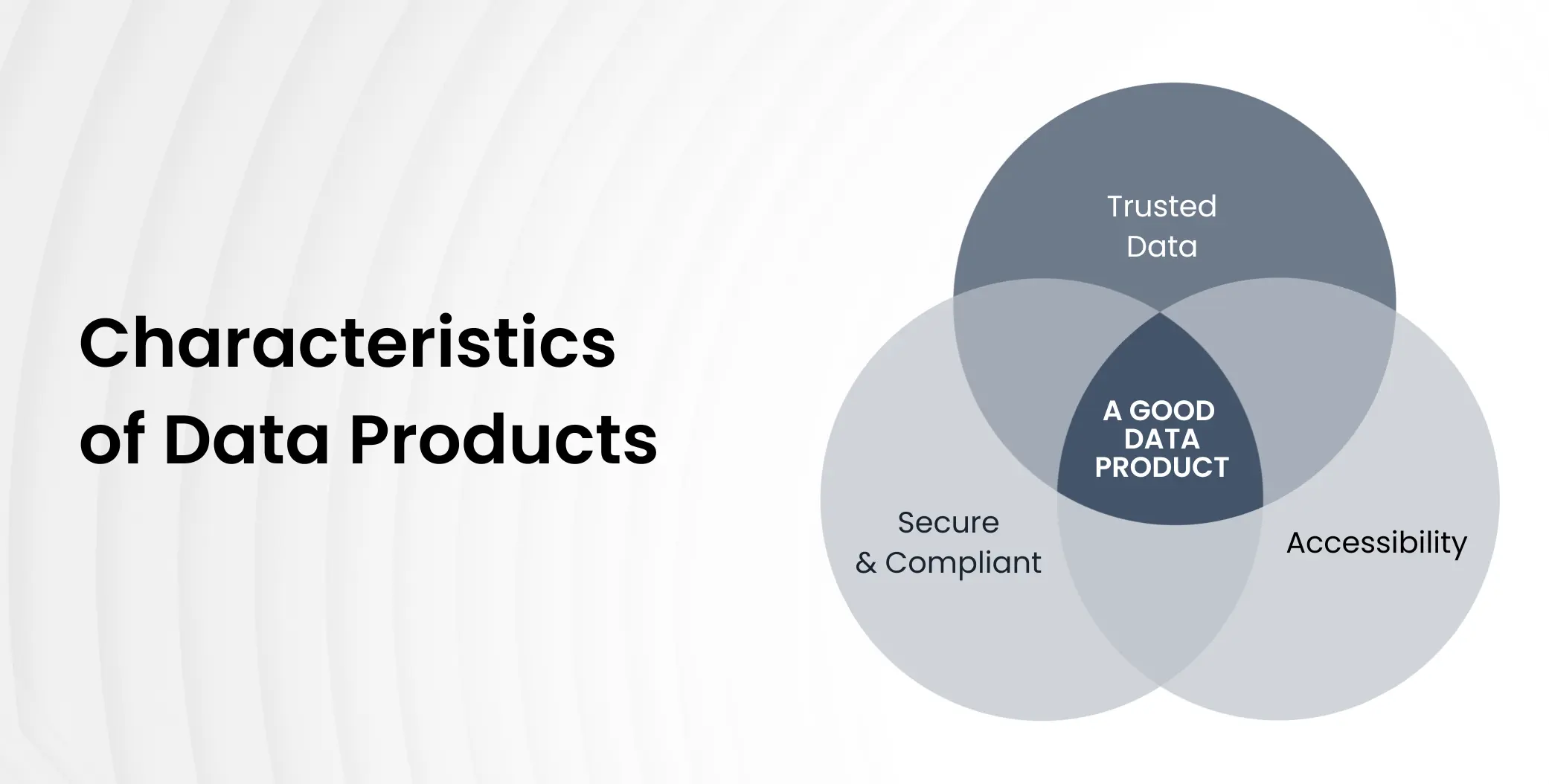
Data Quality Challenges with Data Products
We have seen that one of the key ingredients of data products is trustworthiness & reliability. So it shouldn’t come as a surprise that the value of a good data product is directly related to the quality of its data. Addressing data quality issues for data products means ensuring that data is accurate, reliable, complete, consistent, unique, fresh, and valid. It means ensuring that data is fit for its intended business purpose. Without consistent & reliable data quality, data products will generate faulty and erroneous outputs. Poor data quality issues increase the lack of trust in data products and hinder organizations from enabling data-driven decision-making.
To ensure end-to-end data quality for their data products, organizations must tackle data quality issues at both the data attribute level (frequency, distribution, statistics etc) and at the overall data asset level (volume, schema, freshness). Modern data quality tools ensure end-to-end data quality management by providing both, data observability and quality assurance.
Data observability enables organizations to detect data issues at the earliest and stop bad data from traveling throughout their organizational value chain. Data observability refers to the ability to observe, monitor, and understand the behavior and performance of data systems in real-time. It encompasses the visibility into data pipelines, processes, and infrastructure, allowing organizations to ensure data reliability, quality, and availability. This increases the quality of data products.
Data quality refers to the reliability, accuracy, consistency, and relevance of data for its intended use. It encompasses various dimensions, including completeness, timeliness, validity, and consistency. It provides more granular data and business quality checks to deliver quality data to business users for data products.
Why DQlabs
DQLabs is the Modern Data Quality Platform enabling organizations to deliver reliable and accurate data products for better business outcomes. With an automation-first approach and self-learning capabilities, the DQLabs platform harnesses the combined power of Data Observability, Data Quality and Data Discovery to enable data producers, consumers, and leaders to turn data into action faster, easier, and more collaboratively. DQlabs provides 150+ out-of-the-box business and data quality checks and enables robust data quality and observability measures empowering organizations to deliver successful data products and enable a data-driven culture.