5 Key Benefits of Data Quality and Data Catalog Integration
5 Key Benefits of Data Quality and Data Catalog Integration https://www.dqlabs.ai/wp-content/uploads/2024/07/thumbnail-data-pipeline-blog-24-1024x575.png 1024 575 DQLabs DQLabs https://www.dqlabs.ai/wp-content/uploads/2024/07/thumbnail-data-pipeline-blog-24-1024x575.pngData catalogs and data quality tools are the two core pillars of organizations’ effective data management strategy. Data catalogs enable organizations to discover, understand, and evaluate their data assets for various data and analytics use cases. On the other hand, robust data quality practices ensure that organizations’ data is accurate, complete, consistent, and fresh. This ensures that business decisions are not affected by poor data quality.
However, to effectively manage and harness their data landscape, organizations need to ensure their data catalog and quality tools are interoperable. Before we understand the synergy and integration of data catalog and data quality tools, let’s first briefly understand these components individually.
What is a Data Catalog?
A data catalog provides an inventory of organizational data assets, with effective metadata management, which enables easy search and access to relevant data assets. Data catalogs provide metadata information for data assets such as, what the data source is, when it was created, who the users are, etc. In this way, a data catalog enables easy search and evaluation of data assets for specific use cases.
Manual data cataloging is neither practical nor sustainable. Modern data catalog tools provide automation and augmentation for metadata collection and automated discovery of similar datasets and data tagging. This automation accelerates the cataloging process, reduces error rates, and ensures that data assets are easily accessible for faster-decision making.
What is Data Quality?
Data Quality refers to the reliability, accuracy, consistency, and relevance of data for its intended use. It encompasses various dimensions, including completeness, timeliness, validity, and consistency. Essentially, high-quality data is data that is fit for purpose, free from errors, and aligned with organizational objectives. Clean, accurate, and reliable data is the fuel that powers effective data initiatives.
Modern data quality tools provide out-of-the-box business and data quality checks to ensure data is accurate and error-free. These tools also provide AI and Ml-driven anomaly detection and alert mechanisms for data quality issues for efficient handling and issue resolution. This ensures that organizations’ data is of high quality so data and business teams can use it confidently.
The Synergy Between Data Catalogs & Data Quality
A data catalog provides data and business users a centralized access to organizational data assets. Users can easily locate and explore relevant data assets and add more contextual information such as – business tags, assign datasets to particular domains, etc. This process significantly enhances the business understanding of data assets and increases data utilization.
But this is not enough. Imagine a data analyst exploring a data asset. She finds a dataset with the most relevant attributes for her analysis but one thing is missing – she is unable to assess how good the data is! An analyst would like to see how fresh (updated) this data asset is, whether there is missing data (completeness), how many duplicate rows it contains (uniqueness), and what’s the overall DQ score of the dataset. These are what we call key data quality dimensions. Without these, it would be difficult for a data scientist or any other downstream user to make a decision on whether it is feasible to use these data assets for certain use cases.
That’s precisely why organizations need to integrate their data quality and data catalog tools for effective data management and analytics. This integration would provide several benefits.
Automated quality: With a data catalog, users can add relevant business tags and domains to their data assets, which increases the business understanding of data. Now, here’s what’s interesting – good data catalog tools, driven by AI and ML can detect similar datasets and tags in an automated fashion. This significantly reduces the time to add meaning to the data. If your catalog is integrated with good data quality (DQ) tools, all these business tags can be used as input for DQ tools. Now, the tool identifies that these are similar datasets based on the tags, and applies the DQ rules of the tagged data set to other similar datasets (which the tool itself classifies, based on your tags). This helps to automate the process of assigning DQ rules – you define them once for similar types of datasets, and the rest can be taken care of by the tool.
Enhanced data cataloging: One of the most significant aspects of a data catalog is to enable users to evaluate whether the dataset is fit for the intended purpose or not. This can’t happen without data quality dimensions. By integrating data quality and data catalog tools, users will be able to see the quality metrics and DQ scores in the catalog itself, thereby enabling them to make a faster decision about the usability of the data.
Improved collaboration: Data and business users can easily track, with quality and catalog tools integration, the data quality issues in the catalog itself. The integration will enable users to view the quality issues and alerts in the catalog and notify relevant data personas for issue resolution. This increases collaboration and reduces the issue resolution time as the data users can assign or update relevant data users for relevant quality issues, without having to wait around for users to identify and correct the issues.
RCA with data lineage: Users can access elaborate data lineage and push it to their data quality tools. Data lineage helps immensely to figure out where data looks broken and thus accelerates the process of data quality improvement with effective root cause analysis.
Increased trust in data: The successful integration of quality and catalog tools will empower users with easy access to high-quality data. Users, having clearer visibility of data quality, can now trust their data assets. This increased trust would eventually lead to increased data utilization and data-driven decision-making.
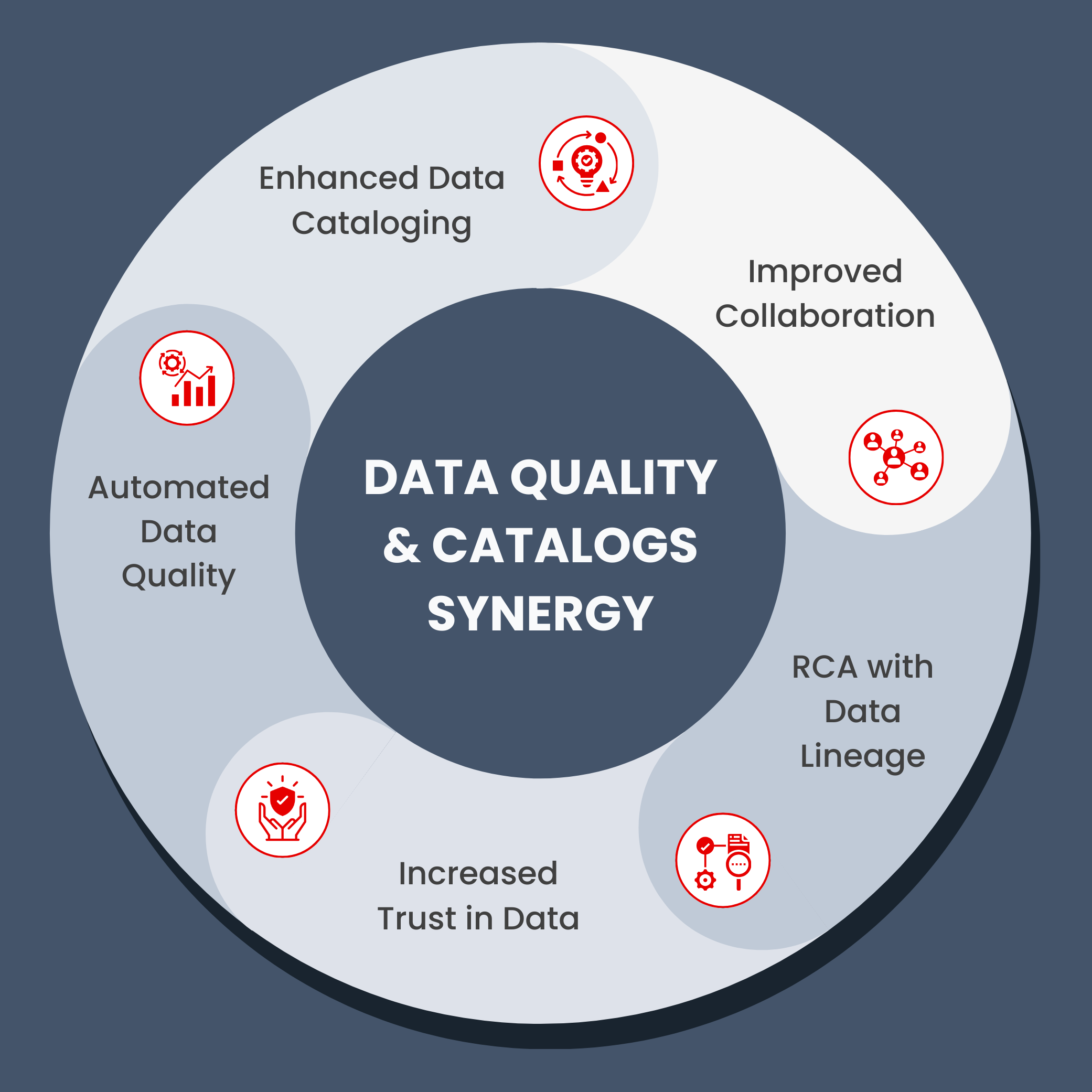
Why DQLabs
DQLabs, The Modern Data Quality Platform, enables organizations to deliver reliable and accurate data for improved business decisions. DQLabs enables successful bi-directional integration with leading data catalog tools and enables centralized access to accurate and reliable data. DQlabs provides the functionalities to push and pull relevant data characteristics to and from various data catalogs.
Push to catalogs: DQLabs provides the functionality to push different data elements such as tables, columns, or domains (a logical grouping of similar datasets) to data catalogs. In this way, users can see all relevant quality measures in their catalogs which will enhance the understanding and usability of datasets. Users can customize their data quality requirements for summaries, alerts, issues, and measures. By doing this users won’t be bombarded with every piece of DQ information and will be able to access what’s relevant for them. This increases users’ trust in their data assets by having a clear visibility of DQ measures.
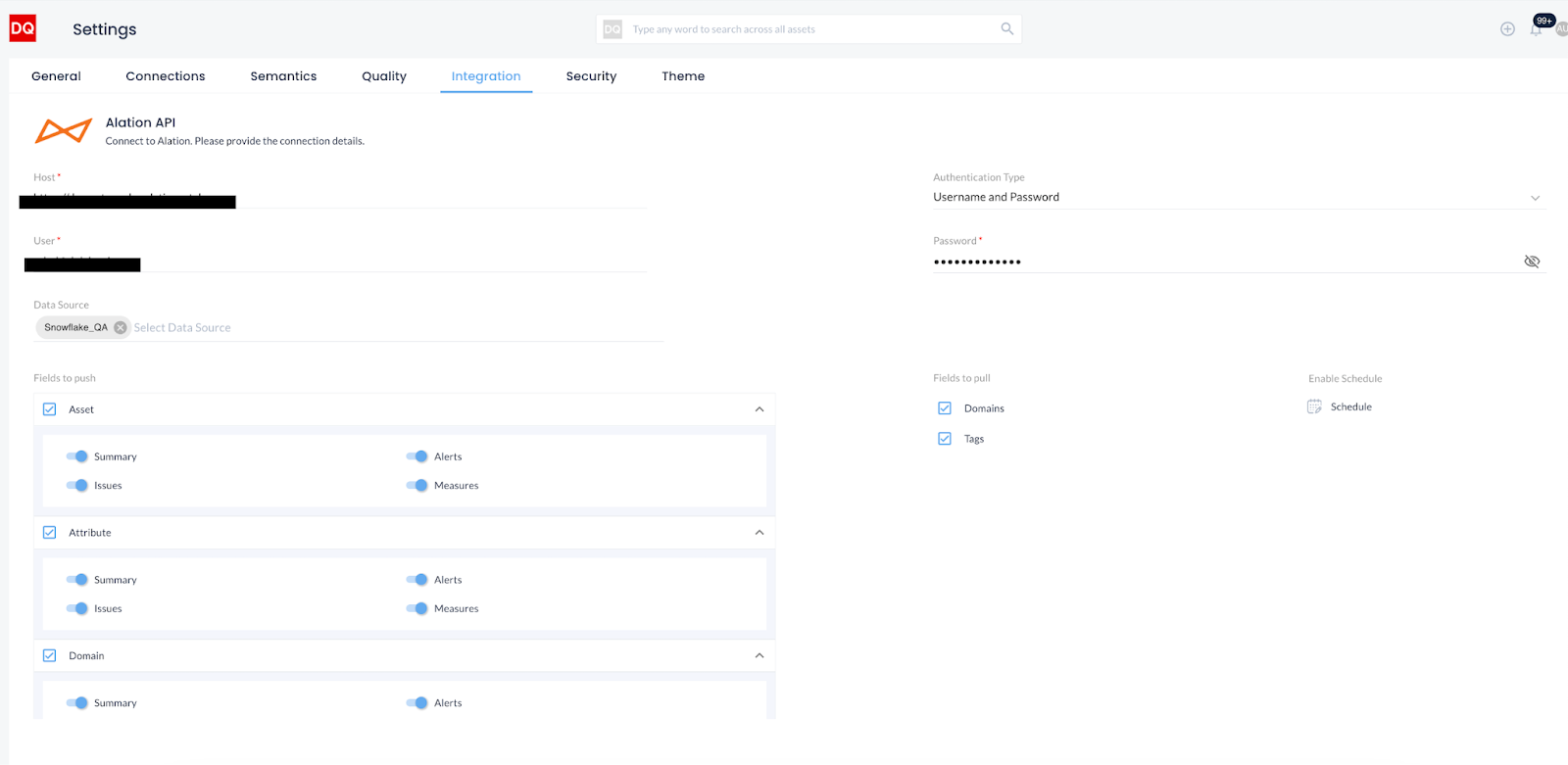
Pull from catalogs: DQLabs has the capability to also pull domains and tags from data catalogs into the DQLabs platform. Domain and tags enhance the usability of data assets by making them more contextual. Domains are logical groupings of similar datasets for specific business functions such as finance, marketing, or R&D. Tags provide more granular information like data types, project name, DQ status, etc. After pulling domains and tags from catalogs, users can apply relevant data and business quality checks on relevant domains and tags. This automates and augments the process of data quality management significantly.
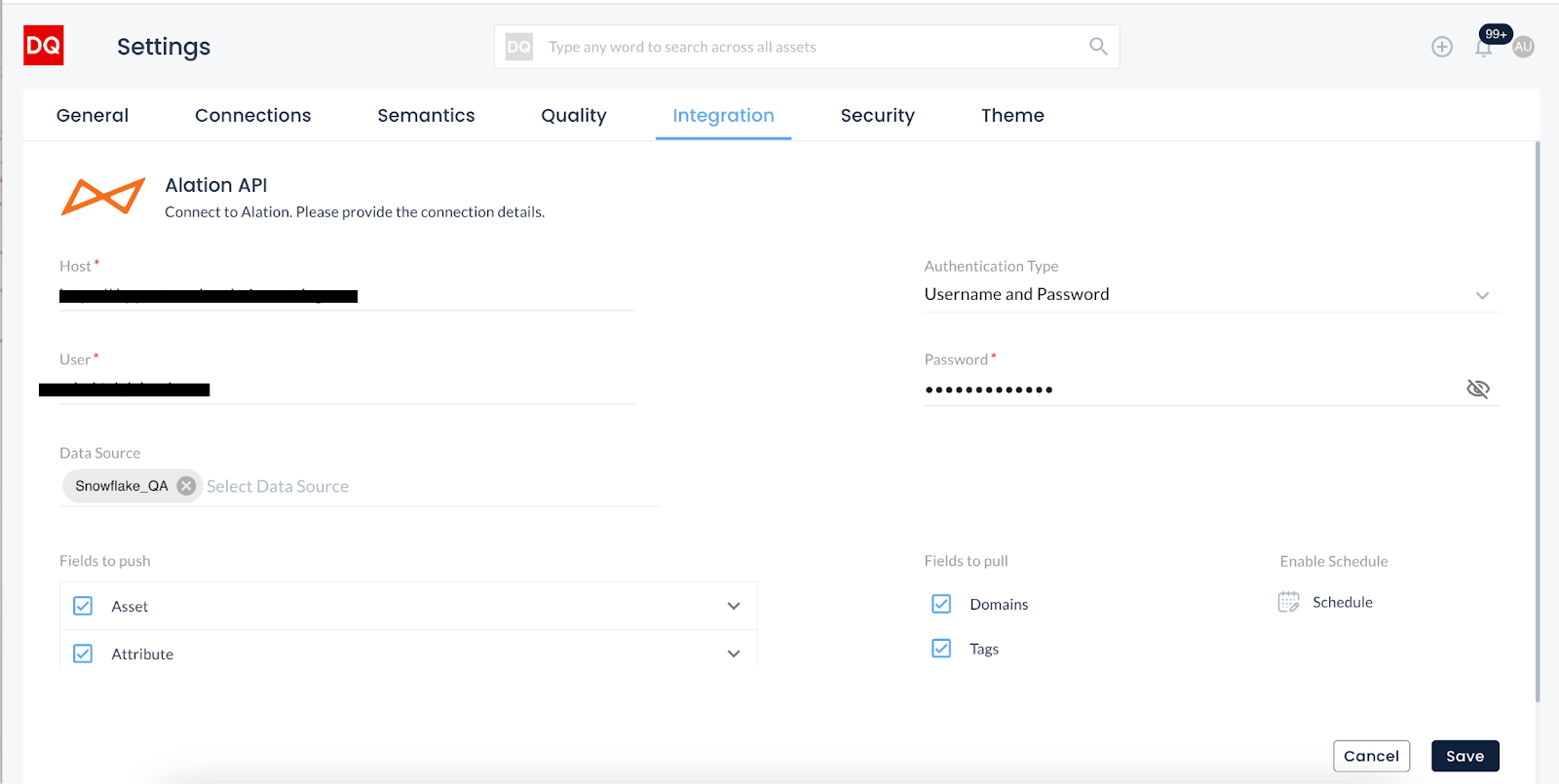
With this integration capability with data catalogs, DQlabs provides high-quality, trusted data to data and business users in their preferred cataloging tools.
Conclusion
The synergy between data catalogs and quality tools is essential for organizations aiming to harness the full potential of their data assets. Data catalogs provide centralized access and understanding of data, enabling users to explore, evaluate, and tag datasets with critical business context. Meanwhile, modern data quality practices ensure that this data is accurate, reliable, and aligned with organizational objectives, fostering trust and enabling informed decision-making.
The integration of data catalog and data quality tools offers several strategic advantages. It automates data quality assessments, allowing organizations to apply standardized checks across similar datasets efficiently. This integration also enhances collaboration by enabling data and business teams to track data quality issues within the catalog environment and update concerned data users. Furthermore, the visibility provided by data lineage facilitates root cause analysis, accelerating the improvement of data quality over time.
DQLabs exemplifies these synergies by offering a modern data quality platform that seamlessly integrates with leading data catalog tools. Through bi-directional integration, DQLabs empowers organizations to maintain a centralized repository of high-quality data with clear visibility into quality metrics and dimensions. This holistic approach not only enhances data trust and usability but also supports organizations in achieving their data-driven goals with confidence and efficiency.