The Convergence of Data Quality and Data Observability
The Convergence of Data Quality and Data Observability https://www.dqlabs.ai/wp-content/themes/corpus/images/empty/thumbnail.jpg 150 150 DQLabs https://www.dqlabs.ai/wp-content/themes/corpus/images/empty/thumbnail.jpgThe Convergence of Data Quality and Data Observability
WHITEPAPER
The Convergence of Data Quality and Data Observability
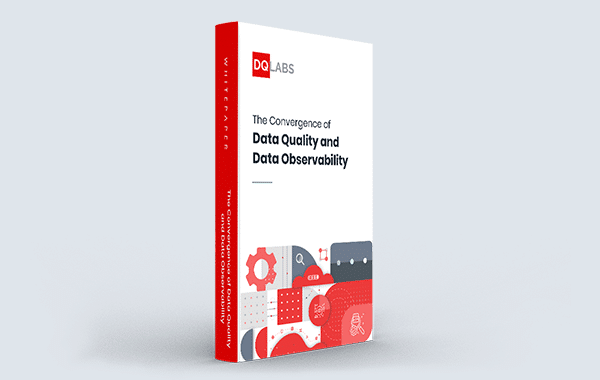
With the growth in data and the adoption of modern cloud architecture or
hybrid with data spread across cloud and on-premise, both producers and
consumers of data are shifting away from the traditional ideologies around
Centralized Data Ownership towards new principles around “Decentralized
Data Ownership.
Instead of flowing the data into a centrally owned data lake or platform, more and more domain owners are adapting the principles of “Data as a product” and serving datasets in an easily consumable way using self-service data infrastructures. Today’s modern data teams use a variety of architectures ranging from Cloud Warehouse to Lakehouse, Data Virtualization to Data Fabrics or Mesh to suit their varying needs of speed and compute power. When it comes to leveraging data for improved day-to-day operations or better Strategic decisions, organizations need to look across both perspectives of minds –Data minds and Business minds as below.
WHITEPAPER
Modern Data Quality Requires a Rethink
It is 2023 and why are we still talking about data quality? Twilight Zone? Deja Vu? Every vendor promises a new fangled approach that they claim is better and will cure all ills of the past. But, we know better.
But, do we?
What if the space of data quality is going through a rethink, driven by the tremendous demands for accurate data from AI and ML use cases? After all, what good do sophisticated models, like ChatGPT, do if they are trained on faulty data? That faulty data may not even be visible to the naked eye. The data itself may be correct, but arrived in the system a tad bit too late or is biased.
WHAT’S INSIDE…
Discover the modernization of the data quality space.
Data Engineers
We look into DataEngineering, which combines three core practices around Data Management, Software Engineering, and
I&O. This focuses primarily on reconstituting data into usable consumer forms by building and operationalizing data
Data Scientists or Data Analysts
While in the world of Data Scientists or Data Analysts aka consumers of the data, the most
common statement one would hear is “Garbage in, garbage out”. In other words, the model
or report developed is only as good as the data that is being fed in. Here the data quality
assessments are defined primarily by the use case and vary for each model and report
generated.
Data Stewards
Now, let’s not forget about the Data Stewards. This role is very confusing in the world of
the modern data landscape and is even turned more confusing with decades of broken
promises from Data Governance platforms.
Data Leaders
Last but not least, Data Leaders aka Business Stakeholders are focused on what
could make their next big win and want to understand how they could use the data on
hand to enable new or improvised strategies toward positive business outcomes.
This clearly shows how business leaders are focused on identifying key business
processes, their KPIs and KRIs, and underlying data or metric assets that have a
direct linkage to organizational mission-critical priorities.
The Convergence of capabilities of Observability and Quality .
In the above triangle picture, the shape triangle in itself depicts the organization hierarchy from top to bottom. On the top are
the leaders making strategic decisions using key vitals i.e., KPI or business metrics to make the next big move while the
bottom layer is the foundational team i.e., data engineers who are creating and responsible for data infrastructure and getting
the data ready for consumption.