Modern Data Quality Requires a Rethink
Modern Data Quality Requires a Rethink https://www.dqlabs.ai/wp-content/themes/corpus/images/empty/thumbnail.jpg 150 150 DQLabs https://www.dqlabs.ai/wp-content/themes/corpus/images/empty/thumbnail.jpgModern Data Quality Requires a Rethink
WHITEPAPER
Modern Data Quality Requires a Rethink
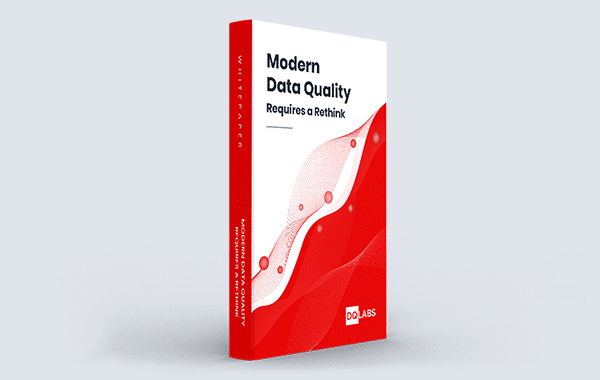
It is 2023 and why are we still talking about data quality? Twilight Zone? Deja Vu? Every vendor promises a new fangled approach that they claim is better and will cure all ills of the past. But, we know better.
But, do we?
What if the space of data quality is going through a rethink, driven by the tremendous demands for accurate data from AI and ML use cases? After all, what good do sophisticated models, like ChatGPT, do if they are trained on faulty data? That faulty data may not even be visible to the naked eye. The data itself may be correct, but arrived in the system a tad bit too late or is biased.
WHITEPAPER
Modern Data Quality Requires a Rethink
It is 2023 and why are we still talking about data quality? Twilight Zone? Deja Vu? Every vendor promises a new fangled approach that they claim is better and will cure all ills of the past. But, we know better.
But, do we?
What if the space of data quality is going through a rethink, driven by the tremendous demands for accurate data from AI and ML use cases? After all, what good do sophisticated models, like ChatGPT, do if they are trained on faulty data? That faulty data may not even be visible to the naked eye. The data itself may be correct, but arrived in the system a tad bit too late or is biased.
WHAT’S INSIDE…
Discover the modernization of the data quality space.
Business KPIs and strategic imperatives
As business teams expand their use of data for new use cases, the stakes are much higher when the data quality lags. Businesses are in a race to leverage data assets faster and don’t want to be slowed down by pesky data quality roadblocks.
The New Rule Book
An organization with 1000 employees, in 2022, has an average of 177 SaaS applications. Most of these applications store data relevant to their needs, However, in order to perform cross-organizational analysis, this data needs to be aggregated, enriched and integrated.
4 Pillars of Modern Data Quality
Perhaps the IT teams would have benefited if the term data quality had never been coined, and instead “business quality” was the goal. In that case, the raison d’être of ensuring data is correct would have been to ensure the business outcomes were being met. In this scenario, the focus shifts from data’s infrastructure to its context.
Benefits of the Modern Data Quality
The goal of a data quality program is to build trust in data. However, trust is an expansive, and often ill-defined term that can include many topics that control and manage data. Trusted data is possible when all the components of the metadata management platform work as a single unit. For example, without accurate data, it is very difficult to ensure that all the data security and privacy programs will work as envisaged.
Case Study
This note examined key aspects and benefits of modern data quality. Many innovative and data-driven organizations are now reaping these benefits. Take the example of Waste Management.
Summary
In a world where so much emphasis has been placed on the speed and agility of analytics, data quality has suffered. However, the modern approach to data quality is once again making it a first order problem, without which modern analytics are rendered incomplete. The focus is shifting from solely checking for technical dimensions like completeness, uniqueness, and integrity to reliability, trust and contextual accuracy.