Are you a data leader struggling to get the most out of your data? Perhaps you’re looking to implement a new AI project that leverages customer data to drive innovation. However, your data team is constantly inundated with requests from other departments, leading to a backlog of your dream data projects.
This scenario is all too common. In organizations where every department, from marketing and finance to sales, relies heavily on data, the central IT team can become a bottleneck for data requests.
Data democratization provides the solution to this problem.
This blog delves deep into explaining the essentials of data democratization and adopting new technologies to assist you in successfully implementing this strategy.
What is Data Democratization?
Data democratization is the process of making data accessible to all employees within an organization, regardless of their technical expertise or role. It dismantles the traditional model where data resides in centralized repositories, controlled by IT gatekeepers. By breaking down data silos and providing easy access to data, organizations empower employees to make data-driven decisions. This approach ensures that data is no longer the exclusive domain of IT and data specialists but is available for use by marketing, sales, finance, and other departments to enhance decision-making, innovation and efficiency.
Data democratization refers to the process of making data accessible to all employees within an organization, regardless of their technical expertise or role.
Importance of Data Democratization
The growing need for data democratization in today’s fast-paced business environment cannot be ignored. It is crucial for organizations aiming to enhance efficiency, productivity, and informed decision-making. As organizations amass vast amounts of data, the ability to access and leverage this data quickly and efficiently becomes a competitive advantage.
By eliminating barriers to data access and comprehension, it empowers non-specialists to view and leverage data effectively, ensuring that the right individuals access the right data at the right time. This process enables employees to make informed decisions and identify opportunities without requiring deep technical knowledge.
Implementing a data democratization strategy often involves self-service analytics tools, allowing employees to use data independently of the IT department. This shift not only improves efficiency and productivity but also enhances employee and customer experiences, leading to increased revenue and greater transparency.
Breaking down data silos and centralizing data storage fosters collaboration and knowledge sharing across teams, improving accuracy and cross-functional decision-making. Additionally, data democratization removes bottlenecks by educating employees on data access and use, allowing the data team to focus on advanced tasks like AI and machine learning.
Challenges of Data Democratization
While data democratization offers immense potential, it’s not without its hurdles. Here are some key challenges that can slow down progress:
- Data Security and Privacy: Ensuring that democratized data is secure and complies with privacy regulations is a significant challenge. As expanding access raises concerns about data breaches and misuse of sensitive information. Organizations must implement robust data governance frameworks to ensure data security and compliance with regulations like GDPR and CCPA.
- Data Quality and Consistency: Maintaining high data quality and consistency across the organization is crucial for reliable decision-making and democratization relies on reliable data. Poor data quality and inconsistencies can lead to inaccurate insights and hinder user trust. Strong data governance policies and practices are essential to ensure data lakes don’t become data swamps, where valuable time is wasted cleaning and organizing data before it can be used for analysis.
- Cultural Resistance: Shifting the organizational culture to embrace data democratization can be difficult, particularly in traditional or hierarchical organizations.
- Data Literacy Gap: Not everyone has the skills to effectively interpret and utilize data. Limited data literacy across an organization can lead to misinterpretations and mistrust of data-driven insights. Investing in training programs is crucial to bridge this gap and equip users with the tools they need to leverage data effectively. But providing the necessary technology and training to support data democratization can be resource-intensive.
- Inaccessible Tools: Complex, technical tools can create barriers to entry. Without user-friendly tools designed for non-technical users, achieving true democratization is difficult as self- serving analytics is a key facet of data democratization. Companies need to reevaluate their data management and analysis tools to ensure accessibility for all.
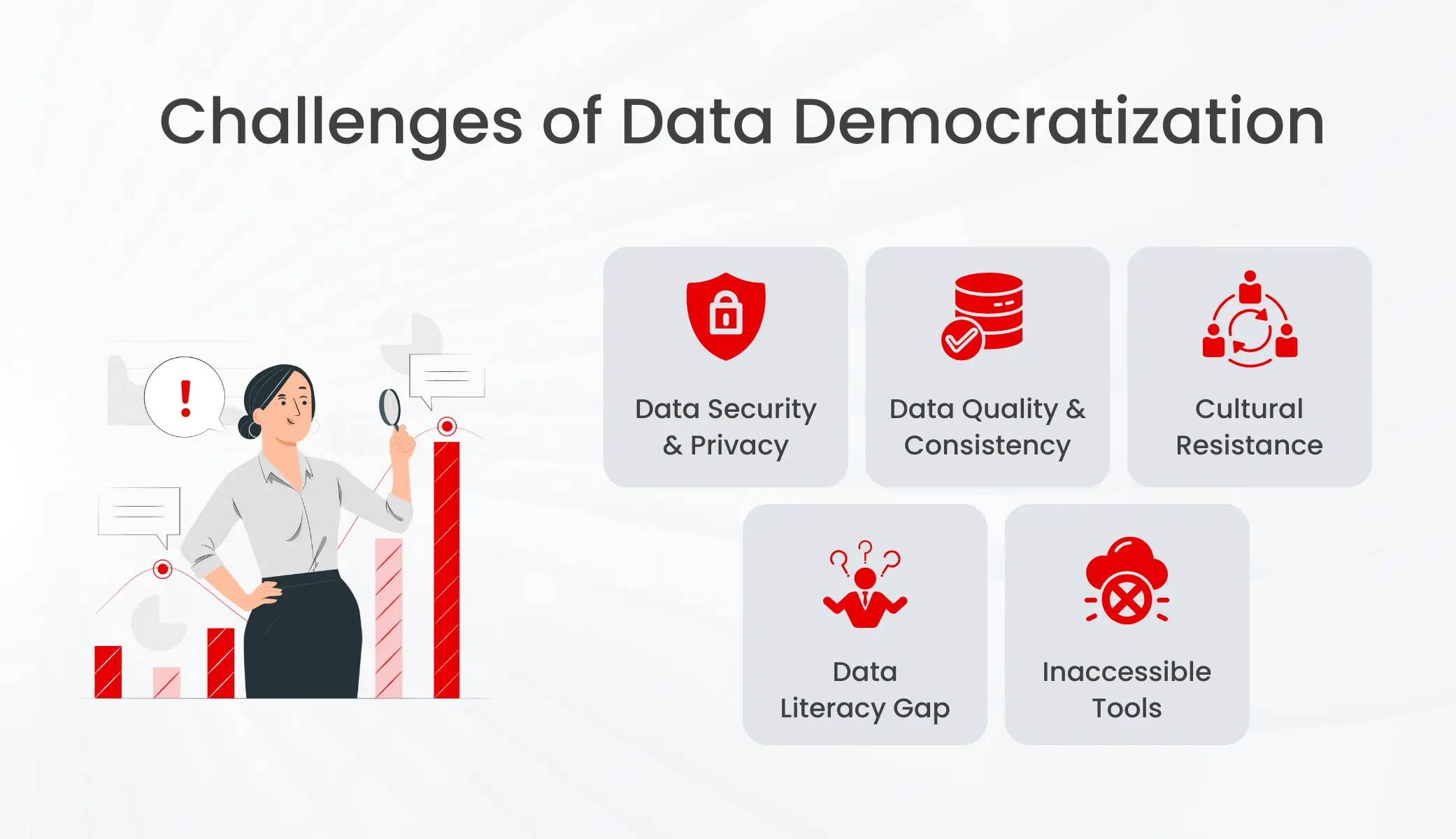
Role of Data Architecture in Data Democratization
The dream of data-driven decision-making can turn into a nightmare if your data architecture isn’t up to the task. While the centralized model offered a single source of truth and improved scalability over siloed systems, it has limitations that become glaring as your data ecosystem grows.
The core issue lies in the lack of flexibility. Adding new data sources, transformations, or analyses requires modifying the entire pipeline, creating a single point of failure and hindering agility. This centralized approach also fosters a misalignment of ownership, where data producers, engineers, and consumers have conflicting priorities. Data engineers focused on ingestion metrics might miss upstream data quality issues, while analysts struggle with unclear or broken data downstream.
These limitations stifle data democratization. The centralized architecture, designed for “data at rest,” is ill-suited for today’s dynamic needs. Data democratization demands a real-time, distributed approach that can handle the increasing volume, variety, and velocity of data. This new architecture needs to be flexible, integrated, agile, and secure to empower users across the organization to derive data-driven insights and implement AI initiatives.
New Data Architectures
To overcome the limitations of centralized architectures, new data architectures have emerged, designed to support data democratization more effectively. Two of the most prominent are Data Mesh and Data Fabric.
Data Mesh
Gartner says, “Data mesh is a data management approach that promotes a domain-led strategy for defining, delivering, maintaining, and governing data products.”
Unlike traditional centralized data architectures, data mesh emphasizes decentralization and domain-specific data handling, ensuring that data products are easily accessible and usable by various data consumers, including developers, business users, and APIs. This approach necessitates clear contracts between data providers and consumers.
To implement data mesh effectively, organizations must develop subject-matter expertise, data management capabilities, and technological skills within each line of business (LOB). This often requires significant organizational changes and the development of roles such as business technologists and citizen data scientists.
The implementation of data mesh is highly flexible and can incorporate various methods, such as creating a marketplace experience for data or subject-specific data marts. Critical to its success is the adoption of a federated data governance approach, consistently applied across domains to support data reuse and shareability throughout the organization.
Core Principles of Data Mesh
Data mesh, conceptualized by Zhamak Dehghani, is built on four core principles that necessitate a fundamental shift in how organizations handle data.
Domain-Driven Data Ownership: This principle assigns data ownership to individual business domain teams, aligning responsibility with the business rather than technology. For instance, in an e-commerce company, finance teams own financial data, and sales teams own sales data. This approach improves scalability, data quality, and clarity of ownership, enabling faster time to market.
Data as a Product: Teams must define not just their own data, but also the data they produce and consume. This involves treating data like a product with clear interfaces, contracts, and access rules, ensuring that data is discoverable, trustworthy, and accessible.
Self-Serve Data Platform: To support domain-driven data, organizations should create a self-service data platform that provides tools for data ingestion, transformation, storage, and analysis. This platform ensures all teams have the necessary resources to manage their data products effectively.
Federated Computational Governance: This principle emphasizes the need for automated governance controls to maintain compliance and data quality. Central governance teams set standards, while domain teams are responsible for their data’s compliance, ensuring scalable and consistent data management.
Benefits of Data Mesh
- Data Democratization: By enabling self-service applications from multiple data sources, data mesh broadens access to data beyond technical users like data scientists and engineers. This domain-driven design reduces data silos and operational bottlenecks, allowing for faster decision-making and freeing technical resources to focus on tasks that leverage their expertise.
- Cost Efficiencies: Data mesh promotes the use of cloud data platforms and streaming pipelines for real-time data collection, moving away from batch processing. Cloud storage allows for scalable compute power and better visibility into storage costs, enabling more efficient budget and resource allocation.
- Reduced Technical Debt: Centralized data infrastructures accumulate technical debt due to their complexity. Data mesh’s distributed architecture, with domain ownership, reduces this strain by improving data accessibility and offering APIs for easier interfacing.
- Interoperability: Standardizing domain-agnostic data fields upfront facilitates interoperability, making it easier for data consumers to link datasets and develop applications that meet business needs.
- Security and Compliance: Data mesh enhances governance practices by enforcing data standards and access controls, ensuring compliance with regulations and enabling comprehensive data audits through embedded observability
Data Fabric
Data fabric, on the other hand, is a data management design concept aimed at simplifying data integration infrastructures and creating scalable architectures. It reduces technical debt by addressing integration challenges and enhancing data utilization, context analysis, and alignment. Data fabrics support diverse data integration styles and use active metadata, semantics, knowledge graphs, and machine learning to create flexible, reusable, and augmented data integration pipelines. This approach is designed to support various operational and analytics use cases across multiple deployment and orchestration platforms.
Core Capabilities of Data Fabric
Seamless Integration and Delivery: Data fabric tackles siloed data by providing methods like cross-platform sharing and CDC (Change Data Capture) to unify your data sources. This virtualized layer eliminates unnecessary data movement, simplifying governance and access control. Traditional data systems like data warehouses can still be part of the fabric as long as they offer standard data access methods.
Metadata-Driven Approach: Data fabric leverages metadata to power its knowledge catalog. This catalog goes beyond traditional offerings by incorporating business context, data lineage, and other details to create a semantic network – essentially a knowledge graph of your data assets. This helps users to explore, understand, and interact with data through a single interface.
Data Governance and Security: Data governance and security reside within the data fabric’s virtualization layer. Permissions for data access, sharing, and modification are all controlled here, eliminating the bureaucratic hurdles often associated with traditional governance practices. This layer acts as a secure bridge between users and the data, ensuring proper authorization before access.
Observability and Transparency: Data fabric prioritizes observability, encompassing data reliability, availability, quality, and security. Additionally, data observability could also incorporate governance aspects, ensuring adherence to access controls and data privacy regulations.
Unified Lifecycle: This covers the end-to-end management of data pipelines, including composing, building, testing, deploying, orchestrating, observing, and managing. Utilizing MLOps and AI, the data fabric ensures a seamless, unified experience for managing data throughout its lifecycle.
High Degree of Automation: Automation is central to data fabric’s efficiency. From managing permissions and data sharing to updating knowledge graphs, automation streamlines various data-related tasks. Infrastructure management can further enhance automation, enabling code promotion and delivery with integrated data quality checks. Data governance is also bolstered by automated tests that identify and report potential security or privacy risks.
Tools like DQLabs goes beyond basic data monitoring, automating data discovery, exploration, and validation. It enables organizations to swiftly identify and verify data at scale, proving essential for those striving to democratize their data.
Automate data quality across the entire pipeline. Try now!
Benefits of Data Fabric
- Enhanced data accessibility: Data fabric enables real-time access to centralized datasets, allowing organizations to retrieve and analyze up-to-date information without reliance on IT support. This improves operational efficiency and enhances decision-making capabilities.
- Seamless data integration: By integrating diverse data sources seamlessly, data fabric eliminates the complexities of data silos. Automated data mapping and transformation processes ensure that data is unified and ready for analysis, reducing errors and improving data accuracy.
- Robust data governance and security: Data fabric provides centralized governance and security controls across multi-cloud environments. It ensures compliance with regulations and protects sensitive information through consistent security measures, enhancing data confidentiality and integrity.
- Scalability and flexibility: Data fabric supports scalability by efficiently managing growing datasets and optimizing resource allocation across various cloud environments. It enables organizations to adapt quickly to changing business needs while minimizing operational complexities and costs.
- Self-service data consumption: Self-service data capabilities empower authorized users within organizations to efficiently discover high-quality data, dedicating more time to exploring data insights that deliver tangible business value.
Implementation Considerations
Here are four questions to consider before implementing data democratization:
- Where is our data currently stored? Determine if your data resides on-premise, in the cloud, or both. Understanding the data storage landscape is crucial for assessing integration challenges and compliance requirements.
- How data-literate are our employees? Assess the level of data literacy across your organization to gauge readiness for data democratization. Consider whether simple assessments or more comprehensive evaluations are needed to identify training needs.
- What are the best-fit data solutions for our needs? Evaluate potential business intelligence tools by reviewing technology resources, seeking recommendations from colleagues, and scheduling product demonstrations. Consider factors like budget, scalability, and vendor support.
- How will we ensure ongoing training and support? Plan for continuous education and support to maximize the effectiveness of your data democratization efforts. Determine strategies for ongoing training and support to ensure sustained adoption and ROI.
These questions will help you prepare a solid foundation for implementing data democratization while addressing key considerations specific to your organization’s needs and environment.
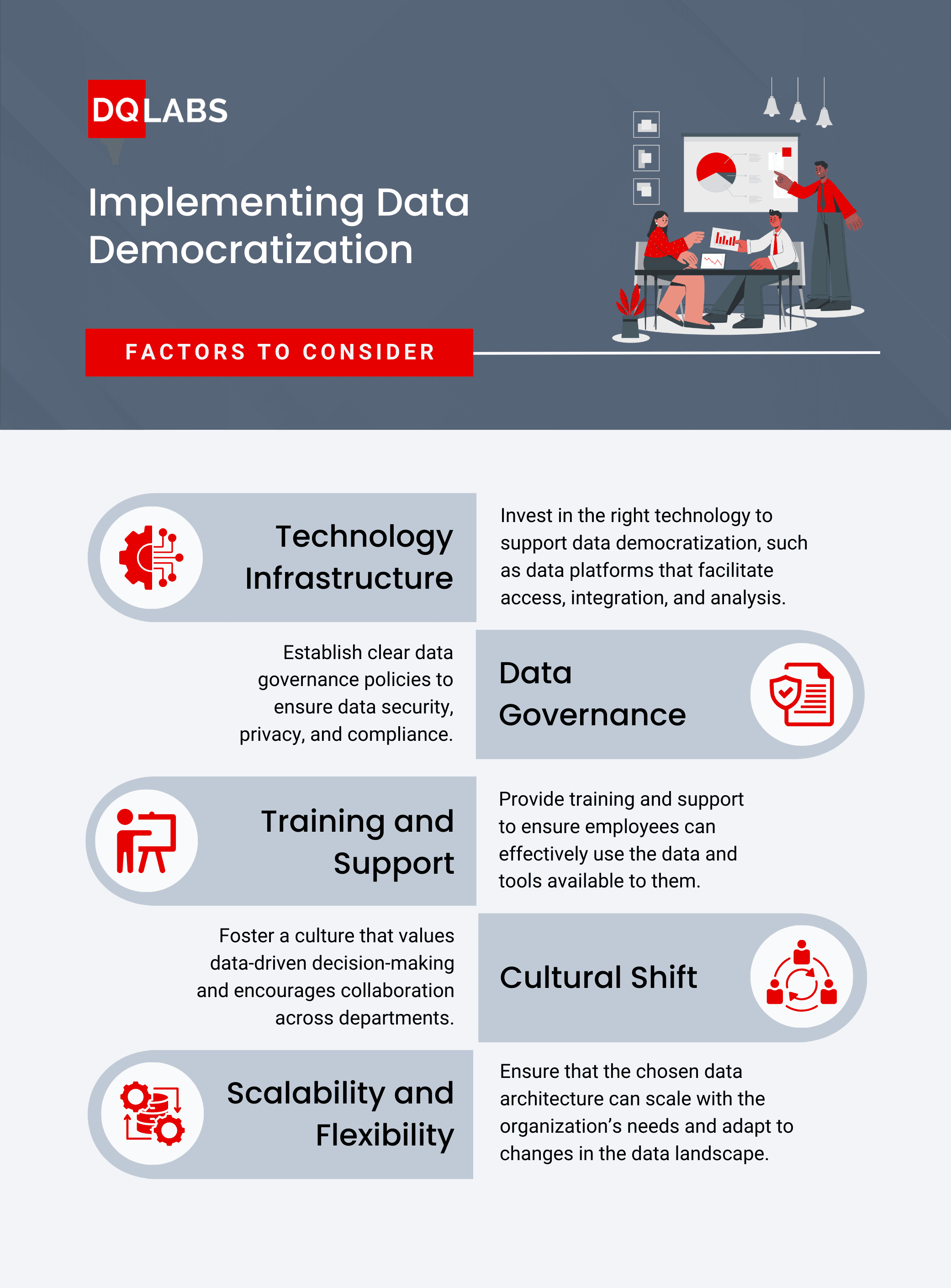
Future Trends
The future of data democratization is likely to be shaped by several emerging trends:
- Artificial Intelligence and Machine Learning: AI and ML will play a crucial role in automating data management and enhancing data accessibility.
- Data Automation: Increased automation in data integration, cleansing, and governance will further support democratization efforts.
- Enhanced Data Security: Advanced security measures will be essential to protect democratized data and ensure compliance with regulations.
- Interoperability: Greater emphasis on interoperability between different data platforms and tools will facilitate seamless data access and usage.
- Real-Time Data Access: As organizations increasingly rely on real-time data for decision-making, technologies that support real-time data access and analysis will become more prevalent.
Conclusion
Data democratization is changing how organizations access and use data, driving innovation, efficiency, and competitiveness. However, achieving true data democratization requires careful planning and the right data architecture. By adopting new data architectures such as Data Mesh and Data Fabric, organizations can overcome the limitations of centralized systems and empower employees with the data they need to succeed.
As we look to the future, advancements in AI, automation, security, interoperability, and real-time data access will continue to shape the landscape of data democratization.